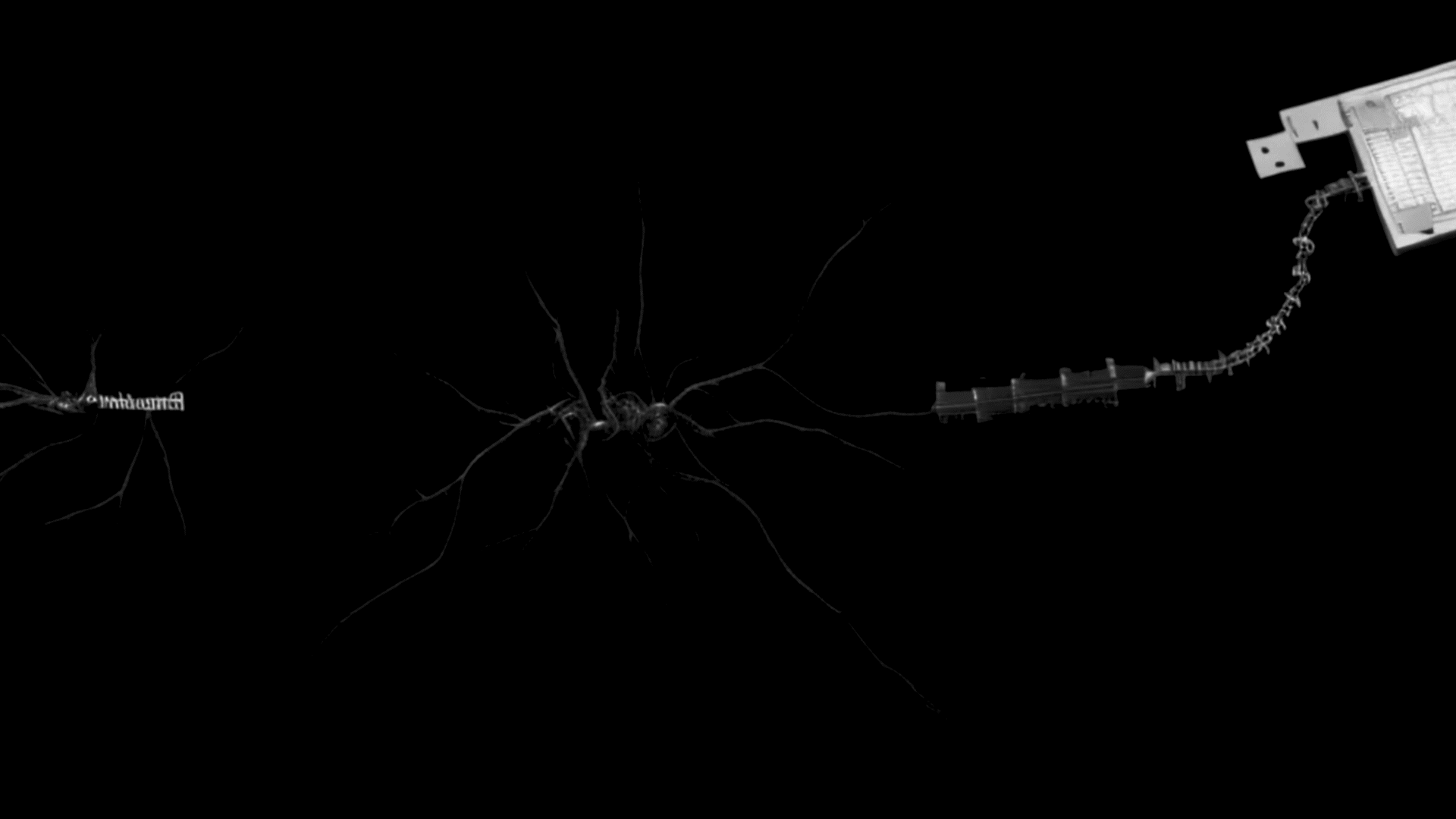
A report by J12, supported by BCG.
Authored by Emmet King
Designed by Maja Shapiro
0. Introduction
What now seems like a short lifetime ago, ChatGPT arrived with such a bang that to have a “ChatGPT moment” has become a turn of phrase. A wow factor, sense of magic, and 100 million users within two months. Excitement created, imagination captured. And the imagination has run, for lack of a better word, wild. A race to build bigger and better models. Unprecedented investment in computing infrastructure to power that development. The quest to apply the technology in every direction. Talk of AGI. Talk of unlocking the solutions to our planet’s greatest challenges. Talk of existential risk and unimaginable threats. Efforts to regulate perceived as both too early and too late. Dollars of investment have poured in. New companies, products, and updates launched daily. Stock prices have soared. Tech CEOs have become rockstars. And amidst this rush, a whole lot of noise as divided opinions, far-reaching forecasts, and heated debate create more questions than answers. A media frenzy of headline after headline.
And then, as time passes, one wonders if the storm is starting to settle, or if it’s only just beginning to brew over the horizon. Previously hyped and hugely funded startups begin to be acquired by the giants they were supposed to unsettle. Others fall to failure. Stock prices and sentiment begin to wobble. More critical questions start to be asked. Where are the revenues? How can such CAPEX investment be justified? Is this a bubble? When will the bubble burst? How intelligent is AI really? What applications actually work? And what can we really expect to see in the coming years?
Gartner might describe this as AI entering into the “trough of disillusionment” in its hype cycle, with adoption, hopefully, slowly picking up at some point from here. Of course, the notion of hype cycles is a somewhat convenient and oversimplified view of how breakthrough technologies unfold - some technologies reach widespread use with a smoother ride, while many others never make it back out of the trough. Thus, given its great promise, it is important to properly reflect on where we are in the development and application of AI as a technology, and how we may impact where things go from here.'
How might the next 10 years look?
If we simplify things, we can look at the future as playing out across a spectrum where at one end we have a continued flow of increasingly powerful AI models, and at the other end we achieve no further model improvements, having already reached peak intelligence, and must rely solely on tooling and better application to drive the realisation of value.
For every future scenario that exists within this spectrum, it’s possible to find an expert that believes strongly in it.
The potential for increasingly powerful intelligence
Given the progression we have seen in model capabilities over the past few years, the investment in compute and infrastructure to drive the development of ever more capable models, emerging model architectures that show the potential to support current approaches, the talent focused on these goals, and the economic incentives at play, there are a number of reasons to feel that there is a decent chance of unleashing increasingly powerful intelligence over the coming years. In this case, the old adage of overestimating what can be done in one year, and underestimating what can be achieved in ten years will ring true like never before, as AI systems reach unfathomable levels of intelligence.
What if intelligence has already peaked?
The other end of the spectrum has the potential to feel like an underwhelming outcome. But in reality, it still represents a decade of transformation and incredible innovation. We’ll see the creation of huge societal benefits, with significant advancements in healthcare, education, and energy systems, alongside increased productivity across a wide range of industries. This is because, even if these already-powerful models do not gain significantly greater intelligence, they are (i) becoming increasingly accessible as size and cost both drastically reduce, (ii) becoming increasingly improved and enabled by infrastructure and tooling related to components such as data quality, knowledge enhancement, orchestration, and agentic workflows, and (iii) becoming more effectively applied due to better-suited UX/UI, integration with AI-first hardware, and the generally increasing maturity of both builders and buyers.
What this report aims to achieve
This report takes a look at all layers of the stack, and the context around it, in order to dive into the themes that are defining the future of AI.
It is our responsibility - as builders, investors, executives, policymakers, or otherwise engaged members of society - to do what we can in order to best contribute to a future in which AI has a positive impact.
In order to inform our view of what the future may hold, to plan for that future and better even shape it, we ask what is next in data and AI?
This report, supported by expert interviews and insights from BCG, analyses the latest technological developments, research papers, investment activity, product releases, and sentiment from the past months in order to form a view on that question. Where we believe helpful, we have included links to relevant sources for further reading.
This undertaking requires us to take a comprehensive look across the entire AI stack - to dive into each layer, consider the dependencies that exist between the different layers, and acknowledge the wider context of factors that impact their development.
We first look to understand the current state of technology - across AI models and hardware - before looking at how it is developing, what capabilities are emerging and may emerge, and what innovations and new architectures may have an impact.
We then look at the current state of the adoption of that technology, considering if, how, and to what extent enterprises are deploying it.
This leads us to identify the major challenges that are inhibiting more widespread deployment, as well as the biggest opportunities for solutions that can address those challenges and thereby enable technological advancement and more widespread adoption.
We then turn our attention to how AI is being applied today, what themes are defining future applications, and where one might look to invest in the application layer.
Finally, we acknowledge the context around the stack and how it impacts the potential pace and scale of AI advancement, considering factors such as regulation, national policy, access to talent and capital, and the impact on the climate.
The Models
The pace of development and magnitude of effort to push the forefront of AI models forward shows no signs of slowing down. Huge sums continue to be invested in companies who set their aims on full Artificial General Intelligence (AGI) or at least commercialising and scaling powerful and highly useful models. Along with investment, ambition, and of course some hype, new models are being released at an unprecedented frequency. Here, we’ll break down the most important recent developments, why they are significant, who is driving them, and what we may expect to see happen next.
In terms of AI lab funding in the past few months…
OpenAI raised $6.6bn at a $150bn valuation
OpenAI co-founder Ilya Sutskever raised $1bn for his weeks-old company, Safe Superintelligence Inc. (SSI)
Mistral raised $640m to fuel their open-source approach to frontier AI
H raised a $220m seed round to build AGI
xAi raised series B funding of $6bn
Cohere raised $500m
In terms of recent model releases…
All the big AI labs have shared significant model releases in recent months, with each of these contributing to the space in various ways. We’ve seen models pushing performance on various benchmarks, others showcasing new capabilities, contributing to open-source development, achieving remarkable performance on a much smaller scale, advancing multimodality, or introducing alternative model architectures. And we’ll dig into these trends and what is significant about each of them in the sections below.
With all of this activity, competition is fierce. And this can be seen in the short amount of time that any model manages to remain at the frontier. In the last six months, a model on average spent only 20 days on top of the LMSYS Chatbot Arena, with AI labs rapidly releasing newly improved versions of their models, or competing labs quickly taking over. Time and again we are seeing new models released that represent what feels like a giant leap forward and away from the chasing pack, only for everyone else to quickly catch up against all odds. This suggests that the breakthroughs of any individual lab positively pull the entire field forward, while also suggesting that few of these labs will succeed over time thanks to intelligence alone - rather, the way in which that intelligence is delivered to users and organisations will increasingly be the key to success.
The graphic below showcases this combination of rapid improvement and high competitiveness, comparing the top ranked models of eight leading AI labs over time.
Given that OpenAI o1 is currently a “preview” it will be interesting to see what further gains are achieved upon the release of further models in the o1 series. While we also wait to see how quickly other models, including those that are open source, are released that bridge the gap when it comes to reasoning and solving hard problems.
The Emergence of Reasoning
Reasoning has long been acknowledged as the next significant breakthrough for AI. For models to evolve from being really good at one-off tasks, and producing one word after the other without really thinking, to those capable of dealing with more complex questions, considering different responses, planning sequences of actions, and predicting the consequences of each.
This is in line with what Sam Altman and OpenAI have outlined as the five stages of artificial intelligence.
According to Altman, ChatGPT achieved level 1 - a chatbot capable of holding a conversation. Now he claims that the reasoning capabilities of OpenAI’s latest model o1 take us to the beginning of the second stage - AI capable of human-level problem solving. In his mind, it has taken a while to make the leap from stage 1 to 2, but one of the exciting things about achieving reasoning is that it should enable stage 3 to come relatively quickly after - where we’ll see AI operating as autonomous agents that can take actions.
Given that OpenAI has positioned o1 as a “preview”, we can expect them to release a more capable version before long. It will now be the task of other model builders to quickly catch up again.
So what makes OpenAI’s o1 model different and special?
While most LLMs consume as much information as possible and use that as the basis to generate new material, o1 can understand different concepts and principles by breaking them down step-by-step. It uses chain-of-thought reasoning and refines its strategies for problem-solving if the current one doesn’t work. In other words, when presented with a problem, it will try out various different scenarios, and, when it arrives at the right answer it receives an internal reward that incentivises for next time, enabling it to “understand” its decision-making, and be aware of mistakes it makes. This all makes it good at tackling complex reasoning tasks and solving problems it has never seen before.
On formal benchmarks, o1 performs very well - significantly outperforming GPT-4o and even expert humans. It appears to be especially capable within areas such as math, coding, and science. When it comes to text-based reasoning, performance does not appear to really raise the bar yet compared to the likes of GPT-4o and Claude 3.5 Sonnet, and given the longer time taken for o1 to respond, for many tasks it need not be the chosen model.
The Role of Open-Source AI
Open source is accelerating innovation and the pace of progress in AI, as researchers and developers freely sharing, collaborating, and building upon each other’s work enables rapid model improvement, reduced duplication of efforts, and a focus on implementation rather than low-level infrastructure. For developers and organisations that need to train, fine-tune, and distil their own models to optimal size, open source AI enables them to do so. It also enables them to avoid vendor lock-in, and protect sensitive data that they would rather not send to closed models over cloud APIs. It promotes transparency and trust as biases and structural issues in training data or model architectures can be identified, and developers can be held more accountable.
Increasingly, there is a vibrant ecosystem of open source models, tooling, and infrastructure propelling AI forward.
Defining open source AI
It is often framed as a battle between closed and open source AI. Where, on one side, we have the closed source players like OpenAI and Anthropic providing access to their AI models without disclosing model architectures, source code, weights, or training data. And on the other side, we have the open-source players including Meta and Mistral, to varying degrees providing public open access, sharing the architectures, source code, weights and training data for anyone to use, build upon, and distribute. Straddling both sides, firms such as Google, Microsoft, and xAI have typically been releasing large closed models, and smaller open versions.
It may be more accurate to describe a spectrum of openness, given that there has been some contention as to whether models that claim to be open, truly are so - with some still restricting access to code and training data. In fact, a group of researchers recently evaluated 40+ models proclaimed by their developers to be open, and assessed them on characteristics related to availability (e.g. source code, training data, weights), documentation (e.g. code, architecture, publication of peer-reviewed paper), and access (to a downloadable package and API). They found that many of the more prominent models are only partially open, or even closed, across most of these characteristics.
Recently, the Open Source Initiative (OSI) - a non-profit organisation formed to educate about and advocate for the benefits of open source - has been leading a process to establish a firm definition of open-source AI. In its current draft form, this requires the inclusion of information on training data and training methodologies, source code, and model parameters.
Is this just an exercise in self-gratification, or why is such a definition important? In short, the traditional open source definition does not account for the additional complexity of AI systems - in terms of requirements for transparency in things like training data and model architecture - and thus, when applied to AI, does not guarantee the freedoms to use, study, share, or modify systems. And, given the great benefits of open source AI development - in terms of promoting transparency and innovation, driving technological progress, reducing costs, and increasing tech availability - the clearer the standards, the more easily more developers can build towards them. At the same time, as regulation starts to distinguish between models released under open source licences versus those that are closed, clear and consistent definitions gain further importance in guiding developers, firms, and policymakers.
Leading open source models
With the dominant strategy for building more capable models being to increase model size, training compute, and thus cost, there has been concern that a fragmented landscape of open source developers would be well and truly left behind. Contrary to the open source software movement - where the time and brain power needed to build solutions like MySQL, TensorFlow, and Kubernetes could be crowdsourced - open source AI requires huge amounts of compute and energy, at a cost beyond the reach of most open source players. However, with Meta going some way to keep their Llama models open, as well as the strong backing of the likes of Mistral, and emergence of somewhat open xAI, open source has three key players with the capacity to compete with the investment of closed source developers. Alongside those players battling for the frontier of large language models, there also exists a vibrant ecosystem of highly performant smaller and more specialised open source models.
The below graphic maps closed vs. open models against the MMLU benchmark. Clearly, two years ago closed-source models were dominating, but in the last 18 months there has been a steep catch-up from open-source developers, with Llama 3.1 really closing the gap at the time of its release. Note that the graphic does not include OpenAI o1, which would represent a leap upwards for the closed models, and it will be interesting to see from here how quickly the open-source players will catch up again.
Some of the most recent notable open source releases:
Meta’s Llama 3.1 - proved to be the most intelligent open model upon its release, while also being the first open model to truly compete at the frontier with the best closed models at the time including GPT-4o and Claude 3.5 Sonnet. And Meta has quickly followed up with the Llama 3.2 collection of models - the largest of which represent Meta’s first large open models capable of processing both images and text.
Alibaba released Qwen2.5 - a family of more than 100 open source models. With each model geared towards different use cases, some are small (500M) and some large (72B), some excel in coding, math, video generation, or game design.
The Allen Institute of AI presented Molmo - a family of open state-of-the-art multimodal models. Beyond its impressive performance, Molmo is noteworthy for being particularly open - publishing both the weights and the dataset, whereas e.g. Meta’s Llama models only share the weights.
Mistral announced Mistral Large 2, showcasing impressive performance for its size - coming in one-third as large as Llama 3.1, but performing similarly on coding and math benchmarks, while also exhibiting competitive reasoning capabilities with the closed source models like GPT-4o and Claude 3.5.
DeepSeek-V2.5 is the latest in the DeepSeek family of models, coming from China. It employs innovative technological approaches, combining a transformer architecture with an advanced Mixture of Experts (MoE) system, enabling impressive efficiency gains while performing strongly against benchmarks.
The Rise of Small Language Models
After years of foundation model providers improving performance by almost entirely focusing their efforts on increased scale, a range of smaller models are showcasing competitive performance, as well as stronger fit for various use cases.
While the massively large foundation models can be expected to dominate generalist use cases, the consequence of this size and general intelligence is that they remain incredibly expensive to run, are faced with reliability issues when applied to domain-specific scenarios, and continue to lack transparency while being vulnerable to security issues and hallucinations. Furthermore, they are also incompatible with computation-constrained environments such as mobile or IoT.
As a result, small language models (SLMs) are emerging to address these issues and fill these gaps in the market, proving to be:
Effective in meeting enterprise needs within specific domains and use cases
Cheaper to run
Easier and cheaper to finetune and customise
Capable of enabling use cases at the edge
Able to operate with lower latency
More efficient computationally, thus also lowering climate impact
Ideal for regulated industries where organisations want to keep data on their own premises
In the beginning of the year, Hugging Face CEO Clem Delangue predicted that 2024 would be a big year for small language models (SLMs), stating.
“My prediction: in 2024, most companies will realise that smaller, cheaper, more specialised models make more sense for 99% of AI use-cases. The current market & usage is fooled by companies sponsoring the cost of training and running big models behind APIs (especially with cloud incentives).”
This has materialised in the shape of dedicated development of smaller models to service various use cases, as well as AI labs releasing smaller versions of their larger state-of-the-art models (e.g. GPT-4o mini offered at nearly 30x cheaper than GPT-4o), allowing end-users to choose between price and performance. With the quality/size ratio of models having been reduced by almost 100 times in just two years, small frontier models can now be stored on smartphones and laptops, putting significant power in the hands of billions of people without any privacy concerns.
Unsurprisingly, much of the drive in SLM development is being led by big tech players like Microsoft, Google, and Apple who:
Provide technology to a great many enterprises - each operating in their own specialised domain and increasingly likely to seek smaller, more specialised models to address those specific needs.
Provide computers, smartphones, and other consumer hardware - all of which need to be running AI on-device.
Some of the most notable recent smaller model activity…
It was Microsoft that first coined the term SLM, when they introduced their initial Phi model in 2023, and earlier this year they released the Phi-3 family of models, and most recently revealed Phi-3.5, outperforming other small models from Google, OpenAI, Mistral, and Meta on several key metrics.
OpenAI released GPT-4o mini, a smaller version of its flagship multimodal model, available at 30x lower cost.
Meta’s open source Llama 3.2 includes smaller language models of sizes 1B and 3B that fit on edge and mobile devices.
Google’s new Gemma 2 2B model, at just 2 billion parameters, delivers best-in-class performance for its size, outperforms much larger models such as GPT-3.5 and Mixtral-8x7B, and is open and accessible. It achieves these outsized results by learning from larger models through distillation.
Apple open-sourced OpenELM, a family of small and efficient LLMs optimised to run on devices.
Nvidia developed Llama-3.1-Minitron 4B, achieving comparable performance to larger models while being more efficient to train and deploy. They used advances in pruning and distillation techniques in order to create the model - essentially starting with a larger model and then removing some less important components of it (pruning), and transferring knowledge and capabilities from the larger “teacher” model to the smaller pruned “student” model (distillation).
Other releases include Hugging Face’s SmolLM, Mistral NeMo which was built in collaboration with Nvidia, various models from Alibaba’s Qwen2.5 family, and Meta’s MobileLLM.
Outside of the efforts of big tech, a few new players are entering the field of small language models, such as Arcee AI, which specialises in domain-specific models and tools for enterprises. A key training technique Arcee utilises is model merging, which allows the combination of multiple AI models into a single, more capable model, without increasing its size, by fusing the layers of different models and taking the best aspects of each to create a hybrid.
Multimodality
The future of AI lies in creating systems that understand our world in all its complexity. If we are to tread the path towards some form of AGI, then we need systems that learn via the sensory input of interacting with the world around them, much like we do as humans. As such, multimodal models have come to the forefront, capable of working with multiple types of data/modalities, processing and fusing information across text, vision, audio, and other sensory data (e.g. related to temperature, motion, or pressure). This empowers AI with greater context as a result of being able to recognise patterns and connections between these different types of data inputs, and thus unlocks more human-like capabilities and the potential to apply AI to a wider array of complex tasks. These capabilities then fuelling the development of general-purpose agents, as well as advanced embodied AI and robotics.
OpenAI’s GPT-4o and Anthropic’s Claude 3 family of models have initially led the way in introducing advanced multimodality. Capable of processing and generating text, images, and other visual information (such as graphs and diagrams), and in the case of GPT-4o also introducing advanced voice capabilities.
A number of new releases have recently pushed this field forward
Researchers at EPFL and Apple published and open-sourced 4M-21, a multimodal model that works with an unprecedented 21 input and output types, including imagery, geometry, text, and the metadata and embeddings produced by other models. Impressively, the model is able to take as input any combination of the modalities it’s trained to handle, and then output any of them. This opens up great potential for far richer use of AI - given the far richer array of information that can be fed to a model in order to prompt towards a desired output.
AI has the potential to learn a lot about the world from the rich temporal data that exists within videos, thus it can be extremely powerful to integrate video within model training. However, efforts to do so have typically been limited to short video clips, given that learning from lengthy video requires models capable of handling millions of tokens in a single sequence - however this comes with significant hurdles related to memory limitations, computational challenges, and the scarcity of suitable large-scale datasets. Researchers at UC Berkeley published Large World Model (LWM), overcoming these challenges (the model is trained to attend long sequences of up to 1M tokens) and capable of advanced understanding of long videos more than an hour in length. This opens up the potential to incorporate extensive video data within model training in order to develop models with a deeper understanding of world aspects and complex environments.
Mistral released Pixtral 12B - its first multimodal model capable of processing images and text - claiming to distinguish itself from other open-source models due to its ability to deliver “best-in-class multimodal reasoning without compromising on key text capabilities such as instruction following, coding, and math”.
Covariant’s RFM-1 (Robotics Foundation Model 1) is a multimodal any-to-any sequence model trained on text, images, videos, robot actions, and a range of numerical sensor readings. Aiming to give robots human-like reasoning capabilities, it can for example combine text instructions with image observations in order to generate desired motion sequences.
Meta launched its Llama 3.2 collection of models - introducing open models capable of processing both images and text.
The Allen Institute of AI presented Molmo - a family of open state-of-the-art multimodal models with performance comparable to other leading models.
2. The Hardware
As Jensen Huang (CEO of Nvidia) outlined earlier this year, we are seeing companies and countries take action in order to “shift the trillion-dollar traditional data centres to accelerated computing and build a new type of data centre — AI factories — to produce a new commodity: artificial intelligence.”
Or in the words of BCG Managing Director and Partner Suchi Srinivasan, “chips are the building block of a new type of economy. No matter what industry you are in—consumer goods, healthcare, shipping—the business processes are becoming AI-enabled."
With demand for AI training and inference continuing to grow as more firms become more serious about generative AI, and as use cases expand to all verticals, everyone needs a supply of AI chips. But, with generative AI workloads being so extremely compute-intensive - requiring significant processing power and memory across the stages of model training, fine-tuning, and inference - the levels of investment in the compute and cloud infrastructure required to train and deploy models has gone through the roof.
With this as the backdrop, we can see the hardware layer as being shaped by a number of key themes that we’ll address in this section:
The intense need for compute - driven by the training of larger models and the demand for faster and higher volume inference.
Unprecedented scale of investment in new data centres and supercomputers in order to secure computational resources.
A new wave of cloud platforms seizing the opportunity to serve an increasingly wide range of compute-hungry firms
An increasing pace of new chip releases and innovations to chip architecture
The Insatiable Need for Compute
Model Training
Over the past decade, as the builders of AI models have sought to push the frontier of performance, the primary strategy has been to increase model size. And this has been highly successful, with Epoch AI estimating that two-thirds of the improvements in language model performance in the last decade have been due to increases in model scale. As Sam Altman reminded us in his recent Intelligence Age blog post, “to a shocking degree of precision, the more compute and data available, the better it [AI] gets at helping people solve hard problems”.
Given this strategy of scaling model size, computational resources used to train frontier models have also grown significantly, with Epoch AI estimating the rate of growth to be 4-5x per year. That itself may be an underestimate, with Mark Zuckerberg in the most recent Meta earnings call stating that the company will need 10x more compute to train Llama 4 compared to what was needed to train Llama 3.
Model Inference
We can highlight three key trends to explain why, in addition to demand to train larger models, demand for compute for model inference is increasing.
Soon enough, almost every interaction that a user has online or with a computer will have a generative AI model running in order to interact, generate content, or complete tasks. And given that humans are more or less online and interacting with computers all the time, there will almost always be a generative AI model being called upon. While some of those models will be running on premise or on-device, many will be in the cloud and thus rely on data centres.
At the same time, AI applications are evolving from chat-based zero-shot prompting interactions, towards more agentic workflows where LLMs are being prompted repeatedly in order to plan, execute sequences of steps, use tools, reflect, improve the output and repeat the process, or otherwise within a system of multiple agents. This leads to the generation of many times more tokens before showing any output to a user, compared with what we have seen in early forms of LLM applications geared towards human interaction and consumption. Thus, faster token generation is becoming increasingly desirable as LLM applications and agentic workflows gain maturity.
OpenAI o1, in proving that a model can perform better on a task the longer it thinks about it, demonstrated a new way to scale compute - to not only add more compute during training, but to scale the compute during inference. This has led to the suggestion that we will see models and use cases where AI systems are set a task and then left to work on it for minutes, hours, days, or even weeks, before providing a solution. This, then, will see the volume of inference compute increase drastically.
So with more applications and user interactions being connected to generative AI models, and with more of those applications generating more tokens per interaction, there is rapidly growing demand for faster and higher volumes of inference.
Unprecedented Investment and Spending on Compute
The big tech players are all investing at an unprecedented pace and scale in massive data centres to fuel their growing AI efforts. Today, there are tens of thousands of GPUs in data centres, by the end of 2025 there will be hundreds of thousands, and in the future we are set to have millions of GPUs in data centres.
According to their Q1 reports, Alphabet (Google’s parent company), Amazon, and Microsoft collectively invested $40bn - mostly in data centres - between January and March this year. While Meta has said that, predominantly as a result of AI-related projects, its capital expenditure could reach $40bn this year.
With no signs of slowing down, all the big tech players have been announcing further huge investments and outlining views of increased need for computational resources. For example:
Microsoft and OpenAI are reportedly working on a data centre to house an AI-focused supercomputer featuring millions of GPUs in a project that could cost $100bn. For reference, that amount is approximately 100 times more costly than some of the largest operating data centres today.
As a result of various data centres planned for its models, OpenAI’s access to compute is expected to increase 8x by 2025 and 20x by 2027.
Amazon plans to spend $150bn on data centres during the coming 15 years as it seeks to retain its cloud computing edge.
Mark Zuckerberg has predicted the development from 50-100 MW data centres to 1GW, in other words of a scale roughly equivalent to the size of a nuclear power plant.
OpenAI pitched the White House on the need for data centres as large as 5GW - essentially city-scale energy sufficient to power three million homes.
Elon Musk’s xAI team built a data centre consisting of 100,000 Nvidia H100 GPUs, with the plan to double its size within a few months.
Microsoft and BlackRock have teamed up to raise $100bn for data centres and infrastructure to power AI.
Oracle announced the largest AI supercomputer in the cloud, with 131,072 Nvidia Blackwell GPUs.
Meta is set to get its own cluster of 100,000 GPUs.
a16z is building a stash of 20,000 GPUs in order to win AI deals and provide access to compute for its startups.
Increasing GPU access
While large tech companies have been able to procure massive GPU clusters for themselves, smaller firms are hindered by a lack of access, as well as being unable to carry the cost of physical hardware investments and the associated risks that come with it - such as inventory shortages or reliance on a single provider. In order to address these needs, a new breed of cloud providers has emerged to provide companies with flexible access to the GPU resources they need.
These emerging cloud providers - specifically focused on hosting AI workloads - provide persistent access to GPU capacity through cloud virtual machines, enabling companies to scale GPU resources up or down according to their needs. They may even facilitate per-second, pay-as-you-go billing to provide companies with even more flexible consumption of GPUs, while some platforms extend their offerings further into the full lifecycle of model hosting.
Some of these players - such as CoreWeave (recently valued at $23bn), Lambda Labs (reportedly raising an additional $800m), and DataCrunch - run a full-stack offering, providing these services on hardware that they own and operate themselves. Whereas others - such as Modal, RunPod, Paperspace, FlexAI, Beam, and Replicate - provide GPU access and services across third-party hardware that they do not own or operate.
European sovereignty
The emergence of these new cloud providers also combines, at least in Europe, with trends towards sovereign cloud computing, as stringent privacy and data protection laws encourage enterprises and governments to seek sovereign clouds that operate wholly within the EU. Providers such as Evroc and DataCrunch seek to meet this demand, while the likes of Lidl spinning out its internal IT unit to compete with AWS et. al is also indicative of a recognition that there is a segment of firms and organisations in Europe that want to be served locally.
Latest Hardware Developments and Releases
Competing with Nvidia’s Dominance
Nvidia’s dominance
Various estimates over the past months have put Nvidia’s share of the GPU market at anything between 70-96%, and although the exact number is unclear, what’s beyond doubt is that Nvidia is dominating the market and has so far been the biggest winner of this AI boom.
So what happens when there is high and increasing demand from the world’s largest tech firms and nation states for a core technology and you are the clear market leader? You become the largest company in the world (at least momentarily), as Nvidia’s valuation soared beyond $3.3 trillion during June. More recently, the firm announced record quarterly revenue of $30bn in Q2, which was a 15% increase compared to Q1, and more than double year-on-year.
Nvidia first developed GPUs for video games in the 1990s, and its chips - excelling at parallel processing of multiple operations - remain the leading solution for handling model training and inference. As Jensen Huang put it in May, “people want to deploy these data centers right now”, and Nvidia’s great advantage is that it has the solution and the scale to meet that demand which shows no signs of slowing down. At the same time, such high demand is giving birth to increased competition from the likes of AMD and Intel, from big tech players (Google, Meta, Amazon) increasing their own innovation efforts as they seek to gain control of core infrastructure, and from a wave of new companies entering the space.
There remains some debate as to whether Nvidia’s stock is a buy or sell, but when Larry Ellison (co-founder Oracle) and Elon Musk are open about begging Jensen Huang for GPUs over dinner, it’s clear that the market dynamics and positioning are favourable. That said, if the world trends towards mostly smaller models - to drive enterprise use cases and on-device AI - there will be increased demand for non-GPU hardware and opportunities for other chip players to compete.
Rising Competition
Recent MLPerf Training benchmarks (measuring how fast systems can train models to a target quality metric) show Nvidia’s Hopper architecture and H100 GPUs to come out on top on all nine benchmarks, beating the likes of Google and Intel, and confirming what was already known - that Nvidia is the market leader.
We will see significant progress in performance from all players going forward, as a space previously characterised by “slower” two-year innovation and release schedules is speeding up, with Nvidia and AMD both announcing product roadmaps with annual releases, which, considering the depth of technology, is quite remarkable. Whether this increased pace of releases represents an increased pace of technological advancement, or if it just means advancement is experienced more incrementally, remains to be seen.
While Nvidia remains very much in the driving seat, established rivals like AMD and Intel are fighting to catch up, big tech players like Google, Microsoft, and Meta are developing their own solutions, and others such as Groq, Cerebras, and Etched have introduced new and competitive chip architectures.
The innovation cycle around AI chips is only just beginning, and GPUs are just the start. As AI workloads mature, we will see the emergence of more specialised and efficient architectures to address these specific workloads, while cost pressure and the need for increased efficiency will also grow as enterprises gain further AI maturity and move from model development to widespread deployment. To oversimplify, GPUs are perfect for training the largest frontier models, but alternative chips may be better placed to address use cases that involve (i) the need for low latency during inference, (ii) smaller low-cost models, and (iii) running AI on the edge.
GPUs, AI Accelerators, and ASICs
Graphics Processing Units (GPUs) excel in parallel processing, making them exceptionally efficient for tasks involving large-scale computations, such as graphics rendering and machine learning. Given their high flexibility and adaptability, they are capable of performing various tasks across gaming, video editing, and AI computations.
For the most part, GPUs retain dominance for a number of reasons including their:
Greater flexibility and easier debugging, making them more suitable when it comes to AI workloads that require adaptations, or during the early stages of AI model development when frequent changes are made.
Capabilities in handling more general-purpose AI tasks, as well as mixed workloads where AI tasks might be combined with other types of computations (such as scientific simulations).
More established developer ecosystems that exist around GPU platforms such as Nvidia’s.
AI accelerators are specifically designed for AI workloads, rather than the more general-purpose capabilities of GPUs. As a result, AI accelerators have the potential to outperform GPUs for AI-related tasks, though the fact that they are fixed in their function and cannot easily be adapted to new tasks, means whether or not they are the better choice depends on the nature of the AI workload and specific use case and requirements.
For AI workloads that require adaptations to new algorithms, GPUs may be more suitable, while they also offer more flexibility and easier debugging during the early stages of AI model development when frequent changes are made.
Application-Specific Integrated Circuits (ASICs) are custom-designed chips optimised for AI workloads, and designed for a specific task (such as running specific algorithms). In this sense, they operate as a type of AI accelerator that is custom-designed for a specific task or algorithm, and as such are highly optimised to offer maximum performance and efficiency for that task.
Thus, hardware developments can essentially be seen to be navigating the trade-offs between providing flexibility versus specialisation in order to provide high performance compute across various AI workloads.
We’ll briefly highlight some of the leading players and the current state of their efforts below.
Releases from the Big Players
In March, Nvidia announced its next GPU, the B200, based on its new Blackwell architecture and due to arrive by the end of this year. The B200 is capable of delivering four times the training performance, up to 30 times the inference performance, and up to 25 times better energy efficiency, compared to its predecessor, the Hopper H100 GPU, and will enable organisations to “build and run real-time generative AI on trillion-parameter large language models”. Not resting on any laurels, Jensen Huang has already announced a next-generation platform called Rubin, in development for 2026.
In April, Intel announced its latest chip, Gaudi 3, specifically designed to power bigger tasks, like training new AI models, and which it claims to be more power-efficient and faster than Nvidia’s H100 GPU. Together with its Xeon 6 processors, which split workloads into different categories for efficiency and performance in order to make data centres more cost-effective, the firm believes it is well-positioned to make AI more accessible for smaller companies and startups, for whom Nvidia’s platform is too pricey.
Part of Intel’s strategy also involves collaborating with other chip and software players, such as Google, Arm, and Qualcomm, in order to develop non-proprietary open software that enables software companies to avoid single-vendor lock-in and retain the flexibility to switch chip providers more easily.
At Computex in June, AMD announced its GPU roadmap and the move towards an annual release cycle. Firstly, coming later this year, the AMD Instinct MI325X accelerators, which Microsoft CEO Satya Nadella highlighted as delivering leading price/performance on GPT-4 inference for Microsoft Azure workloads. In 2025, a series of chips titled MI350 is expected to be made available, based on a new chip architecture and performing 35 times better in inference than the currently available MI300 series. The MI400 series will follow in 2026, based on an architecture called “Next”.
Meta, in its pursuit to catch up with generative AI rivals and retain operating flexibility, rather than relying solely on Nvidia, is investing large sums towards developing its own chips. For now, it’s not replacing GPUs for running or training models, but rather complementing them. While not as powerful as competitors’ solutions, Meta believes that having more control of their entire stack enables them to achieve greater efficiency. The “next-gen” Meta Training and Inference Accelerator (MTIA) is helping to power Meta’s AI products across Facebook, Instagram, and WhatsApp, such as ranking and recommendation ads models.
Google has been developing custom AI-specific hardware for more than a decade, and in May announced Trillium, the sixth generation of Google Cloud Tensor Processing Units (TPUs), which it claims achieves a 4.7x increase in peak compute performance per chip compared to TPU v5e. Google’s models including Gemini 1.5 and Gemma have all been trained and served on TPUs, and the Trillium hardware will train and serve the next wave of Google’s models faster, more efficiently, and with lower latency.
Last year, Microsoft announced two custom-designed chips that would enable it to become less reliant on GPUs: the Azure Maia 100 AI Accelerator, used to train and run AI models, and the Azure Cobalt 100 CPU, designed to run general purpose workloads.
Meanwhile, both Apple and OpenAI appear set to develop their own chips.
Alternative Chip Architectures
In March, Cerebras introduced the third generation of its wafer scale engine AI processor (WSE-3) and server (CS-3). While other chip developers focus on creating tiny hardly visible chips, Cerebras has taken a different approach, with the WSE-3 being 60x the size of any other existing chip. So while the likes of Nvidia utilise GPU clusters to involve multiple smaller chips working together, Cerebras uses a single large chip to minimise communication overhead, reduce latency, and increase throughput for certain AI tasks. As a result, Cerebras excels in workloads that require high memory bandwidth and low latency, such as transformer models, and is gaining traction in more niche markets that require extreme performance for specific AI tasks. Cerebras Inference is capable of delivering the Llama 3.1 family of models approximately 20x faster than Nvidia GPUs and about 2.4x faster than Groq.
Groq’s Language Processing Unit (LPU) Inference Engine is specifically designed to overcome LLM bottlenecks of compute density and memory bandwidth, delivering exceptional compute speed, quality, and energy efficiency on LLMs compared to GPUs. Instead of adapting general-purpose processors for AI, Groq’s Tensor Streaming Processor accelerates the specific computational patterns of deep learning, and this optimised data flow enables a dramatic reduction in latency, cost, and energy consumption. Groq has consistently been shown to be capable of delivering lightning-fast inference speeds, with recent benchmarks suggesting it offers 284 tokens per second for Llama 3 70B, or 3-11x faster than other providers. And the firm recently raised $640m to meet soaring demand for fast AI inference.
Etched is another firm looking to challenge the status quo. Though many players are developing inferencing chips that exclusively run AI models, Etched is unique in that its chips only run a single type of model, namely transformers. Its chip, called Sohu, is an ASIC tailored entirely for running transformers, and as such achieves a streamlined inferencing hardware and software pipeline, able to remove components that are not relevant for deploying and running transformers. As a result, it claims to be able to deliver dramatically better inferencing performance compared to GPUs and other general-purpose AI chips, and recently raised $120m.
SambaNova’s SN40L reconfigurable dataflow unit (RDU) aims to be the GPU alternative, and, paired with the SambaNova Cloud service enables developers to run AI models at unrivalled speed with low latency.
Powering AI devices and running AI on the edge
Until recently, power-intensive AI tools have really only been accessible to developers, but a wave of innovation is bringing built-in AI capabilities to PCs and mobile devices.
At Computex in June, AMD introduced its Ryzen AI 300 Series Processors - a mobile processor with a neural processing unit (NPU) - designed to power generative AI tools and digital assistants on advanced AI PCs, with partners such as Acer, Asus, HP, and Lenovo.
Qualcomm is also bringing its new Snapdragon X Elite chips to AI desktops and laptops, with Microsoft, Acer, and Asus amongst the companies set to release PCs powered by the chips.
Intel Lunar Lake processors will ship in Q3 and be used in the next generation of AI PCs, delivering a performance of roughly three times the speed of the previous generation.
Axelera recently raised $68m as it builds solutions powered by AIPUs (AI processing units) to run computer vision inference workloads on the edge.
SiMa.ai raised an additional $70m for its second generation chipset, specifically built for multimodal generative AI processing on the edge.
Frontier Development
At the frontier of innovations advancing computing capabilities are developments related to technologies such as photonic and quantum chips.
Photonic chips use light (photons) to transfer data instead of silicon, thus overcoming limitations of electronic chips, offering higher bandwidth, speeding up data communication between chips and processing data 100-1,000 times faster than standard chips, with lower energy consumption. Recent mega-funding rounds in companies advancing these efforts include Black Semiconductor (Germany, raised $274m in June), Celestial AI (US, raised $175m in March), and Lightmatter (US, raised $155m in December 2023).
While classical computing uses bits, which can be either a 0 or a 1, as the basic unit of information used to store data and perform computations, quantum bits (qubits) can exist in a state where they are both 0 and 1 simultaneously. Quantum chips then leverage this quantum mechanical phenomena to perform complex computations and solve problems exponentially faster than classical compute. Although still in earlier stages of development compared to photonic chips, and with challenges to overcome related to scalability and error correction, there is great promise. Companies such as IBM, Intel, and Google are continuing to develop quantum processors, while a number of startups and scaleups are working on developing and commercialising photonic quantum computers, such as PsiQuantum (US, raised $620m in a mix of equity, grants, and loans in May), Xanadu (Canada), and Quantum Source (Israel).
3. The State of Enterprise Adoption
With talk of AGI and the promise of all that this powerful technology may be capable of, with lofty expectations for the huge impact on jobs and the economy, with the frequent buzz and hype around new releases and announcements every week (or day), and with company leaders - from CEOs in earnings calls to founders in pitch decks - putting AI at the forefront of what they claim and aim to do, it can be difficult to have a sense of where we actually are when it comes to the usage and adoption of AI. What we do know is that it takes time for a technology breakthrough and polished launch demo to evolve into fully integrated enterprise software, and for workflows and user behaviours to change.
So in this section, we take a look at the data and trends that can help to paint a clearer picture around questions such as:
How are enterprises using and deploying AI?
How are enterprise decision-makers thinking and acting when it comes to AI strategy?
What challenges are preventing greater enterprise AI adoption?
Enterprise Adoption of AI
Enterprise adoption of GenAI is still early but activity is picking up
By the end of 2023, 50% of large companies had launched a genAI pilot, while 10% had started to scale genAI applications across entire functions and/or the overall enterprise.
We look at these numbers and interpret them in two ways. On the one hand, we can see them as indicative of genAI being at a very early stage, with 90% of companies either doing nothing, or still in a pilot phase - and we know how far pilots can be from actual scaled deployment. On the other hand, given that genAI only really came to the forefront at the end of 2022, we can see these numbers as indicating a real intent and fast-pace of development, with 60% of companies already taking action, and 10% already scaling use cases across functions
More recent BCG data breaks down the phases of maturity a little further, and shows an increase in the percentage of enterprises scaling some AI use cases within their organisations. The majority (74%), however, remain in a stage of limited experimentation and proof-of-concept testing, while only 4% of enterprises are considered to have successfully scaled AI solutions across multiple functions and proved a strong readiness for future AI implementation.
Overall, we can see enterprise adoption as being very early - given both technological and organisational complexities - but the pace is picking up with high intention.
This high intention can be further seen by what is happening within AI budgets. A BCG survey of top executives found that 85% are increasing their spending on AI and GenAI in 2024. 89% of executives rank AI as a top three tech priority for 2024, and 54% expect the technology to deliver them cost savings already this year. Similarly, an a16z survey of Fortune 500 and top enterprise leaders found that budgets for generative AI are increasing significantly. In 2023, average expenditure across foundation model APIs, self-hosting, and fine-tuning models was $7m, and with a general sense of optimism and opportunity after promising results from early genAI experiments, spending is planned to increase somewhere between 2-5x in 2024, in order to support deploying more workloads to production.
The same survey of enterprise leaders suggests that, not only are AI budgets growing, but they are increasingly moving from “innovation” spend and other one-time pools of funding, towards being reallocated to more permanent software line items.
And this budget allocation appears to be materialising in actual spending. OpenAI recently surpassed 1 million paid users for business versions of ChatGPT, while Ramp’s Summer 2024 Spending Report shows how the mean accounts payable spend with AI vendors has increased by 375% over the past year, as more companies are committing longer-term to the integration of AI tools within their business critical workflows.
Internal use cases remain ahead of external ones
When it comes to the types of genAI use cases that enterprises are putting into production, we can generally classify efforts as belonging to one of three categories:
Increasing efficiency → taking what is already done but doing it cheaper - reducing cost by automating routine and repetitive tasks
Increasing effectiveness → taking what is already done but improving the quality of output
New opportunity → doing entirely new things that were previously impossible, unlocking completely new revenue opportunities
Currently, most enterprises are occupied trying to implement solutions that relate to efficiency and effectiveness improvements. Very few have the technical maturity, agility, vision, and capable leadership to pursue entirely new opportunities.
For now, it is evident that internal use cases are pushing further ahead than external-facing ones. Internal use cases like knowledge management or text summarisation have gone from experiments to production at a higher rate than more sensitive use cases that retain a human-in-the-loop, such as software development or contract review, and much higher rate than fully customer-facing use cases such as recommendation algorithms or external chatbots. This is intuitive, but emphasises again some of the primary concerns for enterprises when it comes to genAI - related to accuracy, safety, and reliability - which need to be solved in order to enable more use cases to be put into production, and in particular those that expose the experience to the public domain.
A growing divide between executives and employees
70% of workers are excited about the potential of genAI use in the workplace, and 80% want to know how to use AI in their profession, as studies repeatedly show the high levels of positivity that employees have towards utilising AI within their workflows.
But upon implementation, there appears to be more discontent. A recent study by Upwork, interviewing 2,500 global C-suite executives, full-time employees, and freelancers, identifies a disconnect between the high expectations of managers when it comes to the impact of AI, and the actual experiences of their employees when using it. 96% of C-suite executives expect AI to boost productivity, while 77% of employees say that AI has added to their workload and created challenges in achieving the expected productivity gains, and 40% feel their company is asking too much of them when it comes to AI.
Putting these numbers together - with the vast majority of employees wanting to utilise AI, but feeling a lack of productivity gains once doing so - there appear to be continued issues in the successful implementation of AI solutions.
We believe that a combination of the following factors are to blame:
Expectations are being poorly set → AI solutions promise too much, while immature buyers & users aren’t sure what to expect or even what they are buying
Rollout within organisations is poorly managed, particularly in terms of onboarding and training of employees
The technology is not yet good enough for certain use cases, and thus cannot deliver with reliability
The packaging of that technology into product also remains immature, meaning that it is far from seamless for employees to utilise within their pre-existing behaviours and workflows
Firms’ data infrastructure is lacking, such that they continue to be unable to feed AI solutions with enough of the context required to contribute to the job → employees, who have all the context, are left picking up the slack
Klarna: A uniquely well-positioned example
One example of a large company that has seemingly been effective in widespread implementation of AI, with measurable results, is Klarna, the buy-now pay-later and e-commerce giant. The firm claims to have implemented customer service chatbot support that is performing the equivalent work of 700 full-time human support agents, with greater speed and accuracy, and estimated to drive a $40m profit improvement in 2024. AI has been widely implemented within marketing activities and content creation, and across the entire firm 90% of employees are using AI daily in their work, with more than 100 AI-driven projects running across the organisation. In announcing their H1 2024 results, the firm claims that thanks to its AI excellence, it has seen a 73% increase in revenue per employee to $650k over the past year.
Klarna CEO Sebastian Siemiatkowski has openly stated that the firm has stopped recruiting, choosing instead to focus on investing more per employee, and, recognising normal attrition rates in tech firms, expects the firm to shrink in terms of numbers of employees by 20% per year - while growing and becoming increasingly profitable. The firm claims also to have ripped out Salesforce and Workday from its software stack, replacing them instead with their own AI-based workflows.
Klarna is perhaps uniquely positioned to be a frontrunner in applying AI and reaping the rewards. It’s data-centric and entirely digital, old and mature enough to be of a significant size with thousands of employees, but still young enough and agile enough to manoeuvre, and importantly still with its entrepreneurial founder steering the ship. Keeping a close eye on firms that exhibit these kinds of “Goldilocks” just-right characteristics may serve to help us see what effective AI implementation looks like, and what we can expect to see more of across a wider range of companies in the coming years.
Challenges Preventing Greater Enterprise AI Adoption
Enterprise adoption of GenAI is still early but activity is picking up
One 2024 survey of global business decision-makers found 83% of respondents to be optimistic about the potential of AI, but fewer than half to have a program in place to make AI adoption a success, and almost all (92%) harbour some concerns about the risks associated with its implementation, of which cybersecurity ranked at the top. This mix of optimism, lack of preparedness and concern, provides the backdrop and starting point for many an enterprise AI journey.
Once beginning that journey, the path to widespread adoption is long and littered with challenges. Gartner predicts that 30% of generative AI projects will be abandoned after proof-of-concept by the end of 2025, due to issues relating to poor data quality, inadequate risk controls, escalating costs, or unclear business value. We might actually consider this a pretty good number, with a 70% graduation rate from PoC being positive, but it is in any case indicative of the hurdles that an enterprise must overcome.
In UST’s 2024 AI in the Enterprise Survey, 76% of respondents cited a severe shortage of AI-skilled personnel as a key barrier, while 53% of UK businesses admitted to struggling with implementation - often referring to challenges related to security concerns, compliance, regulation, and legacy systems. Meanwhile, in Spain, the lack of an AI framework or policy for use is often seen as the biggest barrier to implementation.
A 2024 study conducted by Coleman Parkes Research found 80% of decision-makers to be concerned about data privacy and security. Though a survey of 100 Fortune 1000 executives by PagerDuty goes even further, finding that 100% had concerns about the security risks of AI, while 98% of those who were experimenting with genAI had paused their initiatives at some point in order to establish company guidelines and policies. The most significant security concerns relate to copyright and legal exposure, handling of sensitive information, and data privacy.
Taking a more technical perspective, the 2024 Retool State of AI Report neatly breaks down the biggest pain points felt by developers, data teams, and leadership within companies ranging from SMEs to enterprise when it comes to building AI applications.
It’s clear that as companies progress along the path from initial pilots and prototypes towards the deployment at scale of robust systems, they encounter a range of challenges. These vary of course between companies depending on each firm’s own set of unique circumstances based on its size, sector, level of data maturity, internal technical competence, intended use cases, existing governance practices, and, not least, the approach of leadership. Despite these variations, it is evident that there are a number of commonly-faced challenges inhibiting greater enterprise AI adoption.
Responsible AI in the Enterprise
As enterprises seek to gain AI maturity, and move along the path from experimentation to scaling applications, greater efficiency and success can be achieved through the establishment of a holistic Responsible AI approach to identifying opportunities and managing risks.
BCG defines Responsible AI (RAI) as “an approach to deploying AI systems that is aligned with the company’s purpose and values while still delivering transformative business impact.”
Put so succinctly, it sounds simple, but in practice, it entails establishing the following, across a large and complex organisation:
Strategy - Comprehensive AI strategy linked to the firm's values that ties back to risk strategy and ethical principles.
Governance – Defined RAI leadership team and established escalation paths to identify and mitigate risks.
Processes – Rigorous processes put in place to monitor and review products to ensure that RAI criteria is being met.
Technology – Data and tech infrastructure established to mitigate AI risks, including toolkits to support RAI by design and appropriate lifecycle monitoring and management.
Culture – Strong understanding and adherence among all staff – AI developers and users – on their roles and responsibilities in upholding RAI.
Enterprises that embed this kind of approach to Responsible AI into their organisation structure, and integrate these practices into their full AI product lifecycle, realise meaningful benefits including accelerated innovation, better products and services, improved talent attraction and retention, and improved long-term profitability.
This may also explain why we observe enterprises in more highly regulated industries to generally be faster in their approaches to GenAI, benefiting from long-established practices related to governance, rigorous processes around monitoring, and data and tech infrastructure that supports them. On the other hand, enterprises operating within industries that have remained only lightly touched by regulation, often lack such structures and are scrambling to create the governance and processes that will enable them to face the new compliance challenges that emerge when deploying GenAI applications.
What this means for startups
For startups looking to sell either AI applications or AI tooling to enterprises, it is key to try to qualify and understand where a potential customer is at with regards to their journey and the establishment of these functions. Trying to sell to organisations that are severely lacking in e.g. tech infrastructure or governance will likely represent time-consuming dead-ends. This view across an organisation may also provide a map of sorts for the various stakeholders that may need to be addressed along a sales process.
Current Drivers of Adoption - Professional Services and Big Tech Partnerships
At this point of the adoption cycle, as enterprises work their way along the process of identifying uses cases, establishing key data and technical infrastructure, mitigating risks, and evaluating performance, it is clear that support is needed, and currently the two paths of least resistance towards greater genAI adoption are:
Professional services firms taking an active role in supporting implementation
The builders of leading technology partnering with established big tech platforms to support integration and distribution
The role of professional services
Given the nascency of commercialisable AI, critical issues related to safety and reliability, and general challenges related to tech transformation and organisational management, it is unsurprising that much of today’s application of AI relies on professional services companies and consultants in order to guide decision-makers and implementation processes.
BCG says that AI consulting will supply 20% of its revenues for 2024, and that this share will increase to 40% by 2026. Christoph Schweizer, CEO, says the firm has “never seen a topic become relevant as rapidly as GenAI”, as they support companies with integrating the technology into their operations and processes, moving from experimentation towards full-scale deployment, while also training board directors and leadership teams.
Similarly, Accenture claims to have booked $2bn in genAI projects so far in 2024. Its latest figures put annualised genAI bookings at $3.6bn, which exceeds OpenAI’s annualised revenue of $3.4bn. While PwC - which claims to be engaged in GenAI with 950 of its top 1,000 US consulting clients, recently announced an agreement with OpenAI, making it the firm’s first reseller for ChatGPT Enterprise. With big money to be made by management and tech consultants, we’re also seeing the emergence of a number of AI-first consultancies, such as FutureSight, positioning themselves as the specialists required to enable firms to reap the benefits of AI.
Big tech partnerships
The other approach to getting AI in production within enterprises, and in the hands of users, has been for the leading labs to partner and integrate with the largest existing tech platforms, enabling them to leverage their existing enterprise presence and distribution. This has long been seen with the Microsoft and OpenAI partnership, but has been replicated by Amazon and Anthropic, Oracle and Cohere, SAP’s investments in Aleph Alpha, Anthropic, and Cohere, and most recently OpenAI’s partnership with Apple.
As these partnerships mature, and integrations deepen, we expect to see a significant increase in the momentum with which enterprises are using advanced AI within their workflows.
The First Wave of Enabling Software
Enablers: Where We’ve Been Investing
What’s Next in the Enabling Layer
Reducing the cost of interference
Providing models with additional knowledge - a focus RAG
Agents have arrived and they need their own picks and shovels
Better AI evaluations will improve and unlock how they are applied
GenAI transforming how software is built and who can build it
4. The Enablers
We define the layers of enabling software within the stack to be the software and tooling that enables the building of AI models and the development of AI applications. They typically either enable (i) entire organisations, or (ii) the developers, AI engineers, and data scientists that are the builders of AI.
We break these enablers down into the categories of:
Data infrastructure
DevOps
MLOps
Generally, we can consider two overarching key questions in order to identify the opportunities for enablement:
What challenges are preventing enterprises from applying AI?
i.e what would enable greater enterprise adoption
As we covered in the earlier section regarding enterprise adoption and challenges, here we consider topics related to:
Ensuring data quality
Handling private & sensitive information
Ensuring model output quality & the minimising of hallucinations
Managing & optimising AI cost & performance
Empowering non-technical users
Adhering to regulations and remaining compliant
Handling risks related to safety & ethics
Etc…
What challenges do developers, AI engineers, and data scientists face within their workflows?
i.e what would enable the builders of AI models & applications to gain efficiency and build more effectively?
Here we consider topics such as:
How to gain efficiency across the process of building, testing, fine-tuning, and deploying applications and models
How to gain increased control over performance & reliability
By considering these questions and pain points, we can then map the workflows and processes within these various enabling layers. Then, in combination with our understanding of the current state of the technology and its implementation, as well as the challenges inhibiting greater adoption, we build a view as to where the most value can be created.
As we saw when looking at the state of enterprise adoption, the vast majority of enterprises are still early in their AI journeys. This means that the key challenges to be solved relate to the initial establishment of core data infrastructure, and the setup of core MLOps infrastructure. We’ll look at each of these a little more closely below.
Establishing core data infrastructure
This refers to the efforts required related to data management, and the implementation of rigorous data governance frameworks and continuous data quality monitoring, in order to ensure that only high-quality and reliable data is fed into AI models. A survey of 334 chief data officers and data leaders, in late 2023, found that the majority of organisations were severely lacking in data preparedness, with companies not yet having created data strategies or begun to manage their data in the ways necessary to ensure generative AI could work for them. 46% of those surveyed identified “data quality” as the greatest challenge to realising genAI’s potential in their organisations. While 93% agreed that data strategy is critical to getting value from genAI, only around a third believed that their organisations had the right data foundation.
Thus, a lot of enablement and investment has focused on:
Data pipeline solutions that orchestrate the ingestion, transformation, and pre-processing of data into formats suitable for analysis and/or as input into models.
Data storage and retrieval solutions necessary for handling unstructured data and powering generative AI use cases, such as vector databases and graph databases. To illustrate this, the latest Retool State of AI Report shows vector database usage increasing from 20% in 2023 to 63.6% in 2024.
Data quality and observability solutions that ensure trust in data, provide real-time visibility across an organisation’s data, and detect issues and anomalies.
In Europe, over the past year or so, this has been reflected in large investment rounds in companies such as Weaviate (vector database, $50m), Vespa (vector database & search, $31m), Qdrant (vector database & search, $28m), and Onum (real-time data observability, $28m).
Setup of core MLOps infrastructure
While MLOps relates to the entire lifecycle of machine learning models - from development and training, to deployment, monitoring, and security - given the immaturity of many organisations, the first wave of enabling software has centred on the initial setup of this infrastructure, and, logically, a focus on the earlier parts of this lifecycle. Simply put, until there are models in production, there is not so much to monitor, so first things first, prepare for the development, training, and deployment of models.
This has resulted in a lot of enablement and investment so far focused on:
Model training and experimentation solutions that organise and analyse information related to training strategies and changes to hyperparameters.
Orchestration and routing solutions that coordinate and manage the sequence of steps required across the ML lifecycle.
End-to-end platforms for enterprise AI, managing all phases of the model and application lifecycle.
In Europe, over the past year or so, this has been reflected in investment rounds in companies such as deepset (platform for building, testing, and deploying gen AI, $30m), Robovision (platform for vision AI, $42m), and Flower (federated learning platform, $20m).
Applying AI to software development
When looking at the potential to enable developers in their workflows, it is clear that the biggest opportunities currently lie with applying generative AI to the processes of writing, reviewing, and testing code. As applications of AI, these of course also belong in the application layer of the stack, but for our purposes we find it useful to gather all of the infrastructure and tooling that empowers the builders of tech, and categorise it within the enabling layer.
Between January 2023 and August 2024, nearly $1bn was invested in AI-powered coding solutions.
In Europe, over the past year or so, there have been big rounds in Builder.ai (AI-powered app development, $250m), poolside (building an advanced foundational model for the challenges of software engineering, $126m plus $500m).
While in the US, we’ve recently seen large investments in Cognition (the makers of Devin, “the first AI software engineer”, $200m), Codieum (AI code generation tool, $65m plus $150m), Magic (frontier code models to automate software engineering, $117m plus $320m), Augment (AI-powered coding platform, $252m), Anysphere (AI-powered coding assistant called Cursor, $60m), and Supermaven (AI coding platform, $60m).
With these three areas considered, the first wave of enabling software can be illustrated by the following “heatmap”.
The First Wave of Enabling Software
Enablers: where we’ve been investing
Data Infrastructure
Validio — guaranteed reliable data with automated data quality and observability.
Deasy Labs — providing the best way to create and leverage metadata within your AI workflows.
DevOps
GitButler — your version control personal assistant.
Rely — internal developer portal providing visibility across the entire software delivery lifecycle.
MLOps
Unify — central platform for optimised LLM deployment, routing any prompt to the best model.
What’s Next in the Enabling Layer
In order to look ahead to the most significant enabling software opportunities going forward, it is important to consider:
What changes as more enterprises gain maturity in their AI adoption, and
In what ways will AI be applied to developer workflows as models improve and more agentic characteristics emerge?
Such that we can adapt our prior overarching key questions to consider:
What challenges are preventing enterprises from applying scaling AI?
i.e what would enable greater enterprise AI adoption scaling?
What challenges do developers, AI engineers, and data scientists face within their workflows that GenAI can address?
i.e. in what ways will GenAI enable the builders of AI models & applications to gain efficiency and build more effectively?
As more enterprises mature, with more organisations beginning to take action, and more pilots transforming into production and fully scaled deployment, the key challenges and focus of activity will also follow this path. What we expect to see:
Core data infrastructural and data quality topics will continue to be high on the agenda to support the scaling of reliable AI in production
MLOps agendas will evolve from predominantly focusing on getting applications up and running (with the focus on e.g. finetuning and deployment), towards topics of observability, security, and compliance
As the landscape of AI models matures, it will become increasingly important to evaluate and orchestrate between the different available models
As AI applications look to take on more autonomous and agentic characteristics, the frameworks and tooling needed to support this will be increasingly in focus
As AI agents become increasingly capable, they will continue to be applied to all parts of the software development process and take a larger share of the workload alongside human developers
Thus, while we’re excited about opportunities across these entire workflows, highlighted below are the topics within which we can expect to see the biggest increases in opportunity and activity, compared to what we’re already seeing today.
Below, we’ll explore some of the key themes that we consider to be driving the focus of the next wave of enabling software.
Reducing the cost of inference
As we covered in the earlier section regarding “the need for compute” in the hardware layer, increased maturity and adoption of AI applications, as well as agentic workflows generating more tokens per interaction, are driving rapidly growing demand for faster and higher volumes of inference. This, in turn, is driving efforts to package and provide the tooling and techniques that can enable organisations to reduce the cost of inference.
Efforts to improve computational performance and reduce costs go beyond innovations in chip design and architecture, and various software approaches can be seen to be having significant impact. Dave Salvator, director of accelerated computing products at Nvidia, has previously shared that the firm typically gets a “2-2.5x boost from software after a new architecture is released”. The usage of different open-source models, shift towards smaller models, fine-tuning, and techniques like batching and quantisation can all be utilised to significantly improve inference efficiency.
Companies such as CentML, Run:AI (acquired by Nvidia), OctoAI (acquired by Nvidia), and Modular have so far been prominent in providing the platforms that enable the cost-efficient scaling of AI applications.
Providing models with additional knowledge - focus on RAG
Approaches to providing models with additional knowledge
The building of AI applications requires that a model’s responses are both contextually and factually accurate, supported by specific knowledge or data sources in order to improve the quality of responses and reduce errors or hallucinations. Providing models with this additional context and information is generally done via:
Prompt engineering
Fine-tuning
Retrieval Augmented Generation (RAG)
Prompt engineering involves the addition of further information within the prompt. This can be effective in some instances, however fails to scale in others due to models having an input token limit. While these limits are continuously increasing with the release of new models (Gemini 1.5 with a context window of 1 million tokens or 750k words), some use cases with a lot of additional data or text information to input may find the limits too low. At the same time, performance of LLMs has typically been seen to significantly decrease as input prompts grow in length. Again, some recent model developments have started to show improved capability of maintaining accuracy and performance even with very long inputs, but for now the degradation of performance is a real hindrance, while longer sequences also imply a quadratic increase in cost (a 2x on the sequence means a 4x in computations, or a 3x increase means a 9x in computation cost).
Fine-tuning involves further training a model on a specific dataset relevant to the domain, in order to adjust model parameters and allow the model to adapt its knowledge. While this is effective, it is more costly in terms of time, money, and computing resources, and relies on having access to the model’s weightings in order to be able to fine-tune them - access which is not available when using closed-source models.
RAG involves combining the generative capabilities of LLMs with external knowledge retrieval from databases, documents, or some other source. By retrieving domain-specific knowledge and context, or up-to-date live and relevant data that is more current than the model’s training data, an LLM can become a lot more specialised. At the same time, RAG provides clear traceability by identifying the sources of information used in generating responses. It can however suffer from some limitations, such as long processing times, and difficulties in handling extended contexts.
As more enterprises move along the adoption curve and gain AI maturity, it becomes increasingly essential that their applications (i) leverage internal data sources, (ii) achieve high performance and accuracy, and (iii) are trustworthy and explainable. While prompt-engineering and finetuning of course have their place, and can be used individually or in combination, we are paying particular attention to developments related to RAG. This is due to the mix of advantages and cost-effectiveness that RAG provides - compared to the limitations of prompt-engineering, and cost and complexity of fine-tuning - as well as due to RAG-specific opportunities we are seeing, which we’ll explore below.
The evolution of RAG
A typical RAG pipeline relates to various areas we have previously highlighted across the data infrastructure and MLOps workflows:
Data ingestion: Ingestion of raw data from databases, documents, web pages or other sources.
Data pre-processing: Processing and transforming ingested data into a suitable format, via techniques such as text splitting, cleaning, tagging, etc…
Embedding generation: Converting processed data into high-dimensional vector representations (embeddings) to numerically capture the semantic meaning of text.
Vector storage: Storing the generated embeddings in a vector database optimised for efficient storage and retrieval.
Query processing: The process of generating a vector representation of a user query and comparing it with the stored embeddings in order to retrieve the most relevant documents or data.
Response generation: Combining the retrieved relevant information with the user’s query and feeding it into the LLM in order to generate the response.
Optimisation of these search and retrieval processes is a constant endeavour throughout the lifecycle of an LLM-powered application, and thus it’s essential to be able to evaluate (i) how information is broken down and pre-processed, (ii) how well the system retrieves information, (iii) trade-offs between speed and performance, and (iv) the use of any other tools or strategies that can improve response quality. As builders of LLM applications continuously strive to improve performance and transparency, we see increased effort going towards optimising RAG pipelines, and subsequently increased opportunities in the tooling and infrastructure that enables those pipelines.
With increasing efforts to optimise RAG pipelines and utilise different methods, tooling has emerged to evaluate RAG applications and support builders in their implementation, such as Ragas, Phoenix, and Trulens Eval.
A range of different types of RAG have emerged, developing capabilities beyond original RAG, and suited to different use cases and needs. These include Graph RAG (using graph-based indexing to more effectively combine and synthesise information), LongRAG (capable of processing larger text units, enhancing performance in extracting answers from large texts), ModularRAG (breaking down RAG systems into specialised reconfigurable components), CorrectiveRAG or CRAG (refines the quality of retrieved documents using an external retrieval evaluator), Speculative RAG, and numerous others.
While the likes of LlamaIndex provide data frameworks for building performant RAG applications, we also see developers being enabled by full RAG-as-a-Service platforms like Ragie, Nuclia and Vectara.
Beyond this, efforts can also be seen to more fundamentally disrupt the approach to RAG, e.g. as seen with RAG 2.0 introduced by Contextual AI, whose founding team was behind the initial research and introduction of RAG in 2020. They see RAG systems today as utilising off-the-shelf models for embeddings, a vector database for retrieval, and a black-box LLM for generation, all stitched together in a way that technically works but is not optimal. By contrast, their RAG 2.0 approach pretrains, fine-tunes, and aligns these different components as a single integrated system, enabling the creation of Contextual Language Models (CLMs) that perform significantly better.
All in all, the need to support models with additional context and information that is accurate, reliable, and transparently configured, is only increasing. And we’re excited to see how efforts in this area continue to evolve and improve.
Agents have arrived and they need their own picks and shovels
We’re seeing a strong wave of AI agent applications increasingly capable of taking on and automating complex workflows. A lot of what is driving this is taking place in the model layer - with frontier models better able to plan and reason. As Sam Altman has outlined, the emergence of better reasoning capabilities in models such as OpenAI o1 provides the foundation for potentially quick advancement to highly capable AI agents that can understand context, plan and reason in order to make decisions, and then take actions in order to achieve goals.
However, improved models alone will not deliver us advanced and autonomous agents, and, as of today, most models remain poor planners and low-level reasoners. This means that most AI agents are still susceptible to errors that become compounded across multi-step processes, meaning they are unable to reliably take on complex end-to-end tasks. As a result, an ecosystem of enabling software and new architectural approaches is emerging that supports agentic workflows through tool use, multi-agent frameworks, chain-of-thought-reasoning, self-reflection, planning, and other methods.
We expect to see this AI agent infrastructure evolve rapidly in the coming years as a result of (i) the abundance of new agent applications being built, and (ii) the changing challenges and opportunities that tooling can address as models improve and agents can become increasingly unconstrained.
We are seeing the emergence of infrastructure and tooling including:
Enterprise platforms for building with agents, such as Emergence
Frameworks and developer kits for building agents, such as LangChain, Oscar, AgentKit (from BCG X), and Semantic Kernel
Platforms for hosting agents, such as LangServe and numerous platforms for enterprise LLM applications
Agent evaluations, such as AgentOps, Braintrust, Langfuse, and Context
Orchestration between different models and agents
Tools supporting the ability to personalise agent memory towards a given user and their current context, such as Letta
Tools supporting the ability of agents to take actions, such as NPi, Mindware, and Imprompt
Tools supporting agents in browsing the web and extracting web data, such as Reworkd, Tiny Fish, Browse AI, Browerbase, Apify, and Browslerless
Authentication for agents to take actions on a user’s behalf, such as Anon and Clerk
Multi-agent frameworks supporting effective hierarchies and collaboration between several specialised agents, such as CrewAI, AutoGen, and AgentScope
Setting of guardrails and constraints to ensure an agent stays on track and avoids harmful or misleading output
Better AI evaluations will improve models and unlock how they are applied
The continued pace of model development, and rate at which new models are released, has increased the focus on trying to understand the capabilities of these models, in which areas they may or may not excel, and how they compare to each other. This is important both from a research perspective - so as to steer efforts and push the forefront in different directions, as well as for developers and organisations looking to take decisions over which models to deploy in which settings.
Standardised benchmarks
A diverse array of standardised tests and benchmarks has emerged, each designed to evaluate specific capabilities, from commonsense reasoning (e.g. HellaSwag or CommonsenseQA), logical reasoning (e.g. MMLU or BBHard), mathematical reasoning (e.g. GSM-8K or MATH), code generation (e.g. HumanEval or MBPP), or question-answering (e.g. MMMU or TriviaQA).
Measuring model performance though, and understanding the capabilities of models in order to compare them in a fair and accurate way, remains a difficult problem to solve. AI benchmarks attempt to evaluate performance in a standardised manner - in simple terms, they essentially set a goal for what an AI model should be able to do - but increasingly fall short in the usefulness with which they do this. Benchmarks are needed that can:
Keep up with the speed of AI development. Where previously benchmarks have been useful in measuring the improvement over time of AI models in achieving a goal, now, as the capabilities of models advance with such speed, they have been achieving near-peak performance on established benchmarks. This leads to a flattening in improvement, as measured by the benchmark - a phenomenon known as saturation - and the benchmark is no longer useful in measuring progress.
Measure generalised capabilities. Where previously benchmarks have been usefully designed to measure capabilities related to specific tasks, now, as models become increasingly generalised in their capabilities - powered by the ability to reason and be creative, as well as the incorporation of various modalities - new benchmarks are required. Although meta-benchmarks have emerged to assess a model’s overall ability by testing performance across a range of tasks, such benchmarks still fall short in managing to capture the full picture of what a model is capable of.
Measure the application of AI in different settings. Where previously benchmarks have been useful measuring progress in controlled settings, now, as models are applied in a range of industries and settings, potentially in unforeseen ways, benchmarks are required that have some appreciation for the context in which a model is being deployed.
Measure more than just performance. Where previously benchmarks could focus on measuring performance, now, as models move from research and labs into applications and broader use, benchmarks are required that take into account the practicalities of cost and latency, while also measuring factors such as bias, toxicity, and fairness, and that can support in understanding the tradeoffs between these.
Avoid being “gamed”. Developers have learned how to game the system and cleverly prepare their models in order to specifically perform well on major benchmarks. This leads to impressive-looking models that are unable to live up to those standards in the wild, outside of the targets of the benchmark “game”. Thus, benchmarks are required that cannot be easily prepared for and “gamed”, and that consequently can measure representative model performance.
It was, for example, these challenges that led Hugging Face to recently launch v2 of their Open LLM Leaderboard, utilising new tests and benchmarks designed to be more challenging and harder to game.
Community-driven leaderboards
An alternative approach is the LMSYS Chatbot Arena Leaderboad - a crowdsourced, open platform, it pits two LLMs’ responses against each other and asks humans to judge which response is superior, using the Elo rating system to provide a community-driven assessment.
Such an approach addresses a number of issues related to standardised benchmarks - a wide range of tasks and responses can be tested, while it is extremely difficult to “game” the assessment of the crowd in the wild. There is, though, the risk that human preference clouds the objective assessment of which answers are better or more accurate, while human preference may also fall short in assessing social factors related to bias or toxicity.
Moving forward
It is clear that in order to improve something, you need to be able to accurately measure it. So increasingly useful and suitable methods of model evaluation will be amongst the most significant AI enablers, given the impact they can have on steering development in the model layer, and guiding the decision-making around how to build with and deploy those models in various applications.
We’re already seeing a growing number of companies entering this space and initiatives looking to address the current shortcomings of evaluations, such as Patronus AI, Galileo, Vals.AI, Vectorview, Scale’s SEAL LLM leaderboards, DeepEval (open-source framework for LLM evaluation), LightEval (open-source evaluation suite from Hugging Face), and Anthropic’s initiative to fund evaluations developed by third-party organisations, with a priority on (i) safety assessments, (ii) advanced capability and safety metrics, and (iii) infrastructure, tools, and methods for developing evaluations.
Meanwhile, a few simple tailwinds suggest that we can only expect to see a greater demand for reliable, customisable, and scalable evaluations that inform the development of both models and their applications.
Fast-improving capabilities of a greater number of models
The increased application of those models in a wider array of settings
The increased scrutiny of regulation
The general desire for trust, transparency, and safety
Thus, we expect to see:
Continued innovation when it comes to broadly evaluating models across tasks, with approaches that can (i) keep up with the rapid pace of AI development, (ii) measure increasingly generalised capabilities, (iii) assess performance in various contexts, (iv) measure practical and social factors as well as performance alone, and (v) remain trustworthy in the face of gamification.
Evaluations that are extremely application specific, rather than assessing overall model capability, evaluating the quality of output within a given task or workflow, e.g. meeting notetaking, customer email summarisation, advertising image creation, etc… In these cases, evaluations must have a strong understanding of what good meeting notes or email summaries look like, and provide guidance as to whether quality is getting better or worse each time the underlying model is tweaked. At the same time, such evaluations need to scale and overcome challenges related to cost and time.
Frameworks and tooling that enable the scalable development of customised evaluations, such that organisations can run their own evaluations, aligned with their specific tasks, goals, and constraints.
Third-party evaluations increasingly performing an auditing role in relation to regulation, or otherwise in relation to generally held beliefs around trust, fairness, bias, toxicity, etc…
GenAI transforming how software is built and who can build it
The application of GenAI to automate at least parts of the process of writing code and building software has been in focus for a while now, since GitHub CoPilot’s initial release in 2021, to the mammoth funding rounds in companies such as Cognition, Augment, Builder.ai, Poolside, Codieum, Magic, Anysphere, and Supermaven. Other currently less well funded but competitive players include Cosine and Lovable. Some of these - like Poolside, Augment, Magic, and Supermaven, are developing their own large AI models for software engineering, whereas others, like Anysphere (and their Cursor product) focus on the developer experience and workflows while remaining model agnostic.
Outside of this set of new players, often the future of DevOps is first seen via the internal tooling deployed within the large tech players, and Google has given us a recent insight into how it uses AI within software engineering. Within Google, LLMs are most heavily applied towards code-completion, with AI-based assistance now completing 50% of all code characters. The next most significant AI tooling deployments are seen in resolving code review comments (where >8% are now addressed by AI), predicting fixes to broken code, and predicting tips for code readability. Google expects to see the next wave of AI assistance to “focus on a broader range of software engineering activities, such as testing, code understanding and code maintenance.”
Of course, the real promise of all this is that within a few years AI-driven software development will essentially enable every human with a computer to develop complex apps and complete products. As a result, products will become increasingly personalised, the entire landscape of how software is built and bought will be changed, and the size and structure of tech companies will look dramatically different. This promise has led vast sums to already pour into this space ($1bn invested between Jan 2023 and August 2024), however we believe that we’re only seeing the beginning of these developments, given that:
(i) The potential value to be gained from these tools is estimated to be huge.
BCG has long estimated that such tools could enable software productivity gains of 30-50%.
Two companies already claiming to be realising significant efficiency gains are Amazon and BP
Amazon, thanks to the use of its genAI assistant for software development Amazon Q, claims to have saved the equivalent of 4,500 developer years of work, with tasks that previously took 50 developer days being reduced to a few hours. This time-saving, alongside enhanced security and reduced infrastructure costs, provides the firm with an estimated $260m in annualised efficiency gains.
BP announced earlier this year that through the use of GenAI and in-house built developer productivity tools they have increased the output of developers by approximately 70% and reduced the usage of external developers by 60%.
Developers themselves expect AI to increase the quality of their code, make it easier to adopt new programming languages and understand existing codebases, generate test cases, and improve code security.
Research looking at the use of GPT-3.5 powered GitHub Copilot for 4,867 coders in Fortune 100 firms found a 26.08% increase in completed tasks, which represents a significant impact from a tool that is now already rather outdated. Another study projects a 33-36% time reduction for coding-related tasks.
(ii) Adoption is fast-paced, but still very early.
According to a 2024 Stack Overflow developer survey, 62% of developers are using AI tools in their development process this year, with 82% of those using AI to write code, while other strong use cases include searching for answers, debugging, and documenting or testing code.
(iii) AI developer tools have significant room for improvement in quality and integration within developer workflows.
According to a 2024 Stack Overflow developer survey, only 43% of developers feel good about AI accuracy, with 31% being sceptical. Only 3.3% of developers believe that AI tools in their workflows are capable of handling complex tasks, with 45% believing that they are bad or very bad at handling them. Currently, most developers see AI as being useful in addressing constrained problems, and “junior developer” work.
The majority of developers observe challenges with AI tools including lack of trust in the output, tools lacking sufficient context of the codebase, and organisations lacking necessary policies to reduce security risks.
(iv) Advancements within models, agents, and tool use, are set to enable AI-based automation of larger scale more complex development tasks.
Devin, OpenHands (previously OpenDevin), SWE-Agent, GPT Engineer, and others have demonstrated the potential of agentic tools that interact with developer environments and other resources in order to complete development tasks. These agentic behaviours continue to be enhanced as models gain improved reasoning and planning capabilities, and as agentic infrastructure improves tool use, querying, reflection, and self correction.
(v) While copilots and AI engineers attract most attention, there are real opportunities for AI to address other specific devops processes.
New tools are automating highly time consuming tasks, or amplifying developer efforts, across domains such as QA and penetration testing, SRE processes, and software monitoring and observability.
(vi) How best to combine AI with human developers remains an unsolved product question.
Depending on the use case, it remains to be seen how best to define where to automate and replace versus where to amplify skilled humans.
The shift towards natural language as the interface for software engineering tasks is still early, and it remains to be seen how best to design the ideal human-computer interaction in this context.
The Next Wave of AI Applications
Themes Defining the Next Wave of AI Applications
It doesn’t need to be generative: finding the right AI mix
User experiences will never be the same again, but we still don’t know what they will become
AI-first consumer hardware is shining a light on where B2B software may go
5. The Applications
There has been much talk over the past couple of years about the potential that AI has to be applied widely across different verticals and address the pain points and workflows of all sorts of organisations and end users. It has been forecast, as a result, to drive a revolution of unprecedented productivity gains and subsequent societal benefit.
But now, questions are being asked as to where those applications are, and if they will be able to deliver on that promise. Given the huge amounts invested in the layers further down in the stack, all eyes are on the application layer to confirm that the technology can be transformative, and that all the infrastructural compute capacity will be needed in the years ahead.
Despite the flurry of application building activity, we believe that we’re still more or less somewhere between day zero and day one. As we’ve seen, AI models are still gaining important capabilities, tooling and infrastructure enabling those models and connecting data to them is also early, and thus the overall level of adoption is immature, which means that much has yet to be tested and figured out with regards to how, when, and where AI is best applied. Drawing some parallels with the shift to smartphones, where some of the biggest applications to emerge were not built until years after the platform shift, we can be sure that many of the big AI applications of tomorrow do not yet exist.
Throughout this section, we’ll look at the changing shape of AI applications, the themes defining the next wave, and how we as an early stage investor define our interest in the application layer.
The Next Wave of AI Applications
The AI applications of the past couple of decades can typically be characterised as focusing on classification, regression, clustering, and anomaly detection, in order to learn patterns in predominantly structured input data. With the aim then to make predictions, forecast events and behaviours, and to make decisions or provide recommendations. This has led to the creation of a range of solutions, but generally more so in relatively data heavy and digitally mature domains, with examples such as music recommendation, inventory planning, targeted advertising, demand forecasting, or credit risk analysis.
With the emergence of LLMs and generative AI, we have increased capabilities to leverage vast amounts of unstructured data (often estimated to account for 80-90% of all data), and by learning the underlying patterns and distributions of data can generate entirely new content and output.
Typically, this first wave of genAI applications has been dominated by those that can be characterised as:
Suiting a prompt-based workflow
Text-to-text, text-to-image, text-to-video, image-to-video, voice-to-text, text-to-music, etc…
Content generation across various workflows and tasks
Involving the analysis of large amounts of unstructured data
Creating knowledge hubs by bringing data and documents together from various sources and making it possible to search and query
Summarisation and analysis of complex documents
Transcription of audio
Occasionally leveraging additional tools to complete tasks
Utilising e.g. web search or retrieval-augmented generation (RAG)
Completing actions on third-party applications, e.g. bookings, purchases
With the first wave of genAI applications, we have seen significant activity across domains and workflows such as:
Content creation in media & marketing
Automation of interactions within customer service, sales, general email
Analysis & creation of complex documents, e.g. within the legal profession
Transcriptions of work meetings, medical appointments, or other interactions (think AI scribes of various types).
We’re now seeing a number of emerging and advancing capabilities in the model layer that are propelling us towards a next wave of genAI applications that are capable of taking on a larger share of more complex tasks. Some of the notable developments include:
Models with improved reasoning, planning, and memory
Increasingly multimodal models, capable of understanding, combining and outputting different types of data and content
Frameworks enabling the creation of more autonomous agentic workflows
Although we’re not yet at a point of fully autonomous agent applications in production, with the right frameworks and constraints AI is gaining increased agency. With this, we start to see the emergence of a greater number of applications capable of providing significant value within mission critical workflows, as genAI applications develop from being supportive junior colleagues to owners of tasks and, in some cases, entire workflows.
Beyond these technological advancements that lie mostly in the models and tooling around them, we are also seeing developments in the product design for how we as human users may most effectively interact with intelligent systems. UX and UI are increasingly becoming AI native and changing what a good user experience looks and feels like, while we’re also seeing the first releases of devices and consumer hardware that are AI at core and further enable next-gen applications.
In this chapter, we’ll shine a light on some of the developments and themes that we see to be defining the next wave of AI applications.
Themes Defining the Next Wave of AI Applications
As outlined, technological advancements in the capabilities of models, improved tooling around those models, and next-generation UX/UI and devices are all pushing the application space forward and evolving what AI looks like in production. In this section, we’ll further explore these and other themes that are central to how AI is being applied now and in the future.
It doesn’t need to be generative: finding the right AI mix
In previous sections, we’ve explored how technological capabilities have evolved from traditional predictive ML towards generative AI. The hype and excitement around genAI has attracted an understandably great deal of attention and energy to explore what the technology may be capable of and how it can be applied - and it makes some sense that developers have rushed to hack and experiment, and that enterprises have put their focus on exploring opportunities in order to not to get left behind.
In some cases, though, builders and enterprises have been thinking too much “genAI first” and hunting for whatever opportunity to apply the technology, rather than astutely identifying opportunities where genAI should be applied. Not only does this rarely work, but it can also be at the cost of funnelling attention away from ML approaches that could be highly useful. Increasingly, CIOs have made clear the pressure they feel under to shoehorn genAI into areas better served by other forms of predictive AI, or even simply a spreadsheet.
Meta is one example of a large organisation that established a specific GenAI group during 2023, but then in the beginning of 2024 decided to merge it with its long-established Fundamental AI Research (FAIR) advanced AI research division. Google also last year merged its two AI labs Google Brain and DeepMind, to form Google DeepMind. The conclusion: it’s more effective to bring expertise and advanced technology together to create solutions, rather than to develop technology in silos and see from there what it can solve.
And it’s not only at the enterprise or advanced AI lab level that this is the case. The hype, FOMO, and curiosity around genAI has also led some early-stage companies or solo builders to focus on the technology’s potential at the expense of the potentially best solution.
So now, as the dust starts to settle, we’re seeing more instances of a deep understanding of problems, workflows, and what needs to be solved, and thereafter how technology can play a central role in doing so. With that lens - being problem-first and technology agnostic, rather than genAI-first and seeking a problem - we see more mature approaches to the application of AI, where more traditional predictive ML is utilised alongside genAI in order to deliver the greatest value.
For example, a revenue management solution may use ML to predict which customers are most at risk of churning, use another model to recommend the best-suited content and timing of delivery for each customer, and then generative AI to create the personalised messaging and visuals.
Increasingly, applications are combining predictive ML for analysing data, forecasting, and making predictions, with generative AI for interpreting unstructured data, synthesising large datasets, and creating new content. The different forms of AI can interact with each other in various ways, depending on the application. For example, AI models may sequentially feed one another, so that one model’s output becomes another model’s input, or they may communicate iteratively, where a continuous cycle of feedback and reinforcement mutually enhances the output of each.
Agents, agents, agents
As outlined earlier when looking at the waves of AI applications, we are seeing significant activity in the development of AI agents that aim to be capable of handling complex tasks, with increasing autonomy. In short, agents are AI systems that can understand context, plan and reason in order to make decisions, and then importantly take actions in order to achieve goals. By allowing agents to interact with software, databases, online services, APIs, and digital tools, they are enabled to automate all sorts of workflows, cognitive tasks, and essentially a lot of what makes up “knowledge work”.
Today, as models are still fairly poor at planning and only now gaining greater ability to reason, agents are susceptible to errors that become compounded across multi-step processes. As a result, most agents remain unable to reliably take on complex end-to-end tasks, and the best implementation is to constrain them to narrower use cases with fewer steps required to complete tasks. Setting these constraints effectively, we have seen a flurry of activity with applications deploying AI agents within:
Sales & marketing → automating lead generation, personalised outreach, and initial sales conversations
Content creation → generating social media content, writing articles, and creating videos
Software development → writing code, building applications
Progress is fast though, and there continues to be rapid improvement in what AI agents are capable of → aided by (i) new models such as OpenAI o1 that exhibit stronger reasoning capabilities, (ii) structured outputs enabling models to better interact with third-party software via APIs, and (iii) real-time APIs enabling almost no latency in speech-to-speech interactions. In addition, there continues to be a wave of enabling software and new architectural approaches that support agentic workflows through tool use, multi-agent frameworks, chain-of-thought-reasoning, self-reflection, planning, and other methods.
From text-based agents to multimodal agents and multi-agent workflows
Many of the initial agent applications have been heavily text-based, however, with increasingly advanced voice, vision, and multimodal models, alongside the further “unlocks” mentioned above, AI agents are being greatly enabled to come closer to the kind of human-level problem-solving that typically requires the use of multiple senses and context gained from understanding the world across text, vision, audio, and a range of other sensory data. This has already led to a rise in agents specialised in other non–text modalities, such as:
Computer vision based agents - that learn from human actions across software by recording screen actions and using computer vision models to replicate the specific steps → ideal for automating system-based, complex workflows that a human user might typically complete within and across various software applications.
Voice agents - where we are seeing the replacement of many existing phone interactions (e.g. customer service), replacement of existing conversations (e.g. therapy, coaching, tutoring), and where we expect to see the emergence of entirely new kinds of conversations that aren’t currently taking place today.
The other next progression in agent applications that we can expect to see more prominently is the building of multi-agent workflows. Rather than singular agents being tasked with handling ever-increasing complexity, applications that organise a team of agents each specialised to excel in a specific area, and capable of effectively collaborating, promise to deliver even greater value. It is the effective collaboration that is the key to these multi-agent systems creating value rather than chaos, and with better tooling and frameworks that effectively define how to distribute the work between agents, how they should communicate with and direct each other, how they should correct each other, which models suit which tasks, etc… we should start to see more multi-agent systems applied effectively.
Big tech agents
Beyond startups offering new agent applications to automate knowledge work, various big tech players have been launching agents of their own to support their customers.
Oracle recently announced 50+ role-based agents to help organisations reach new levels of productivity across HR, supply chain and manufacturing, enterprise resource planning, and customer experience.
Salesforce launched Agentforce - a platform enabling businesses to build and customise their own AI agents, e.g. across customer support, sales outreach, sales coaching, and marketing campaign management.
Workday announced new AI agents to transform HR and financing processes, including a recruiter agent and an expenses agent.
ServiceNow introduced AI agents to enhance productivity by orchestrating workflows, integrations, and data across a business.
Service-as-Software
As we’ve seen with the exploration of agentic AI, and the potential for intelligent systems to take on a large share of complex tasks, making decisions with autonomy, we see a change in the role of software.
Previously, in the paradigm of Software-as-a-Service, a company selling software would be selling access to its platform or product, and the customer or user would be responsible for using that product and its tools in order to complete tasks and achieve a desired outcome. Typically, when it comes to services, it is the company selling the service that takes responsibility for the completion of work and achieving a desired outcome. For example, a marketing software may help you to plan and manage your marketing campaigns, or a marketing agency might take that on and do it for you. A financial software may help you with accounting, invoicing, and expense management, or an accounting firm may provide the service to manage everything for you.
AI application companies are now leading us towards Service-as-Software, where instead of providing access to the tooling, they provide the full service. foundation capital has written thoughtfully on this topic, referring to the phenomenon both as Service-as-Software, as well as Software-as-Autonomous-Service.
Automation of work is not a new phenomenon, but the potential for AI agency and autonomous decision-making significantly increases the scope of work that software applications may be able to handle, as they start to take on the responsibilities of highly skilled workers. And as the scope of work increases, so to does the budget that these applications tap into - going from tapping into a software tooling budget, to tapping into a budget equivalent to the salaries a company is currently paying it’s employees.
This then represents a massively greater market opportunity, as shown in the figure below. A company such as Workday generates annual revenues of $2bn for providing enterprise HR tooling, which is dwarfed by the $200bn that companies spend globally on HR salaries. The overall size of this enterprise AI automation opportunity is estimated to be $4.6 trillion → combining $2.3 trillion in annual salaries across HR, security, software engineering, and sales & marketing, with $2.3 trillion spent on outsourced services and salaries. Fully autonomous agent applications will be going after a huge chunk of this.
Of course, we’re not yet at full automation, and in many workflows it’s unlikely that we will be for quite some time. Thus, new applications have to exist somewhere between the paradigm of Software-as-a-Service providing access to tooling, and Service-as-Software providing full automation and a complete service. Those that can best thread the needle between the promise and limitations of autonomous AI, target the relevant workflows that are ready to be automated away, position themselves well amidst the sensitive debates within enterprises regarding human-in-the-loop versus full replacement, and offer products and pricing that support this transition, have an unprecedented opportunity ahead of them.
Professional services as software
Some of the biggest AI application activity so far has been within professional services, with, for example, large funding rounds in legal AI companies such as Harvey and Leya. Companies such as these are providing incumbent professional services firms - in this case law firms - with AI-powered applications and workflows in order to increase efficiency and improve quality of output. Similar approaches can be seen across other professional services categories including tax and accounting, management consulting, and financial services
While the starting point for most builders of new applications seems to be to offer something to the incumbent professional services firms - which makes sense given those firms already sit on the end customers, have a high need to increase efficiency, and have a high willingness to pay - we are excited to see the emergence of more startups that aim to be the AI professional services firm, compete with non-technical incumbent firms, and address end clients directly.
The current state of both technology and market/customer sentiment (it still feels safer to have a big-brand human advisory firm), means that we are unlikely to see full-scale purely AI law firms, AI tax advisory, or AI management consulting, for some time, if ever. However, we believe strongly that there are opportunities to identify specific use cases and offerings to particular target customers, offer those directly, and compete them out of the hands of the large traditional firms. In other words, while we may not see the AI management consultant yet, we might see AI-powered cost reduction analysis for SMEs, or AI-powered risk management. While we may not see the full AI law firm yet, we might see AI-powered patent applications and trademark registrations, or AI-powered business formation and structuring.
The more narrowly these use cases can be defined, the more easily they can be automated and thus applications can drive a price-point far below that which traditional professional services firms can offer. And some of these use cases, although narrow, will represent sizable market opportunities.
User experiences will never be the same again, but we still don’t know what they will become
As AI applications are evolving - gaining agency, taking on larger shares of workflows, redefining those workflows, changing the role of human users within those workflows, and enabling new forms of interaction between human users and intelligent software via chat, voice, or visuals - we are seeing a huge shift in user experiences, and entirely new ways to think about UX and UI.
We are yet to see exactly what the winning modes of interaction design will be, but those applications that manage to navigate these changing dynamics in the best way possible, with a deep understanding of the workflows and users they impact, will have the advantage in winning and redefining their categories. Along these lines, OpenAI recently launched Canvas, as the first major update to ChatGPT’s visual interface since its launch two years ago, noting that “making AI more useful and accessible requires rethinking how we interact with it”.
Some of the questions that builders of applications need to think about and design for, while considering the specificities of their context and use case are:
To what extent should AI be visible and in the foreground? Should it merely play a role in providing value under the hood, or should it be present and ready to be “asked” for support?
What is the level of transparency and explainability that should be shared with the user, and how and when should that be shown? Is it important that a user should be able to see how the AI is “thinking” or the steps it is taking, or what has guided a given outcome? If so, is it important for the user to see such information while actions are being taken, or is it sufficient for them to be able to do so after the fact?
How to design for latency and the time it takes for AI to complete a task, especially if that task was initiated or prompted by a user who awaits its completion. How can this waiting period be designed such that it doesn’t feel slow or cumbersome, or even such that the waiting period can be useful? This question continues to evolve now as reasoning models like OpenAI o1 take the time to think about their responses, and pave the way for future instances where AI models may be left to work on a task for minutes, hours, days, or even weeks, before providing a solution.
How can you embed a supportive AI agent in the context of the given task and workflow, such that it is helpful to the user as they are working and taking actions, rather than being a resource they call upon on the side of their activity. Or in other words, the difference between having a teammate or colleague that is doing the work with you, versus having to stop your work in order to get guidance from a smart coach on the sideline.
What is the balance for how much the user expects the AI to know and thus execute things on its behalf, versus how much user input should be required to support the AI in handling things? When can AI be allowed to “guess” and then perhaps be corrected later, versus when should it ask for clarification in order to better inform its approach? How can AI be provided with sufficient context so as to allow the user to express their intentions with minimal effort?
What are the optimal modalities of interaction? Typically we have interacted with software by clicking around, writing in fields, or writing code. LLMs have enabled more text-based chat interaction design, but we can also see how some applications may be designed around more voice interaction (e.g. with OpenAI’s Advanced Voice Mode, and an enhanced Siri within Apple Intelligence). One recent development that may begin to further define how we interact with AI has been Anthropic’s release of its Artifacts feature, evolving Claude from a purely conversational AI to a more collaborative work environment, allowing users to manipulate and refine AI-generated content in real-time, or easily incorporate it into ongoing projects.
In what instances will we see applications become more “headless” as they gain their context from various data sources and are less reliant on having fields completed by a user, or any conscious user input.
Going further with entirely dynamic generative UIs
As we move forward, we will soon start to see more dynamic software such that generative UIs will be created for the user based on what is needed or preferred in that instance. In other words, evolving from the questions we have predominantly been asking here of “what is the right interface and interaction design towards AI?” Towards asking “what data and context do we need to provide AI so that it can best decide what interfaces and mode of interaction to serve?”
Coframe is one example of a tool that uses genAI to dynamically optimise website or app content. As a16z ponder, “in a world where the UI is adaptive to the user’s intention, interfaces could become just-in-time composition of components through a simple prompt, or inferred from prior actions, rather than navigating through nested menus and fields.”
AI-first consumer hardware is shining a light on where B2B software may go
During the past year, we have seen the development and launch of a range of new AI-first consumer hardware, as efforts are made to reinvent what our devices should look like, how we should interact with them, and what they should offer us. The primary driving forces being that:
If we have highly capable audio and visual AI, we don’t need to only interact with software via touchscreen but rather we can interact through voice or visual signalling.
If AI is capable of inputting large amounts of ambient data such as the visuals from our surroundings, or the audio from our conversations, we need new devices that can easily be worn in the most suitable positions for this data collection, rather than sitting only in our pockets or on our wrists
Some of these initial efforts flopped immediately upon launch, and others have yet to be released, but among the new wave of consumer devices we have seen:
Humane’s Ai pin - a multimodal device designed to act as your assistant and second brain as it can listen in and capture your surroundings.
The wearable AI pendant from Limitless, providing personalised AI powered by what you’ve seen, said, and heard.
The Rabbit r1 pocket companion.
Friend’s neck-worn device that listens to you in an effort to combat loneliness.
The PLAUD NotePin - acting as your wearable AI memory capsule, ready to record and take notes.
IYO ONE AI earbuds that enable you to talk with all different kinds of audio applications powered by agents.
Various big tech players are also innovating when it comes to consumer hardware:
Spectacles - Snap’s AI-powered smart glasses.
Meta’s Orion augmented reality glasses.
Midjourney recently announced that it’s “getting into hardware” and started recruiting to its hardware division.
Copilot PCs arrived earlier this year and are already getting upgrades, with Microsoft introducing new experiences that streamline daily tasks and empower users with new capabilities
The launch of Apple Intelligence brings smartphones into the AI era, providing access to on-device models and private compute models, as well as a highly improved Siri.
Google has similarly sought to bring new AI features to Android, with the new Pixel 9 giving a glimpse into the next wave of AI-powered smartphones.
New user interaction design is typically first seen in consumer applications and behaviours, so it is with a keen interest that we follow developments in this space, confident that the products that start to show usefulness to consumers will set the tone for future B2B application development. As the cost of hardware decreases, and edge AI is increasingly efficient, we can expect to see the emergence of more vertical B2B hardware-enabled applications.
Robotics and embodied AI
New multimodal models that aim to give robots human-like reasoning capabilities are advancing and enabling a wave of new robotics companies, and paving the path from single-purpose robots pre-programmed for specific tasks, towards general-purpose robots capable of adaptive learning for many tasks and situations.
Amongst the recent activity in frontier foundation models for robotics, we have seen:
Covariant’s RFM-1 (Robotics Foundation Model 1)
Physical Intelligence developing foundation models to power robots
Skild AI’s Skild brain - the first scalable robotics foundation model
World Labs - the spatial intelligence company building Large World Models
While OpenAI earlier this year rebooted its robotics team in order to supply models to other companies building robots
And we are seeing increasingly impressive examples of general-purpose humanoid robots such as Figure 2 by Figure AI, NEO by 1X Technologies, Phoenix by Sanctuary AI, Atlas by Boston Dynamics, Tesla’s Optimus, and MenteeBot by Meetee Robotics.
In addition to improved spatial AI and robotics foundation models, a number of other trends are enabling the field of robotics. Methods such as video learning, human imitation, and simulations are increasing data collection for training, while robot components such as sensors, cameras, batteries, and motors have all greatly improved while becoming far cheaper. At the same time, there is a growing ecosystem of tooling and infrastructure supporting key components of the robotics tech stack across hardware, data processing, system software and integration, control, intelligence, safety and compliance. While we’re also seeing more open toolkits making robotics more accessible, such as HuggingFace’s LeRobot.
For most use cases, we do not expect to see general-purpose humanoids addressing needs. Rather we expect to see a continued development of single-purpose or special-purpose robots applied to areas such as healthcare (e.g. surgical assistance, monitoring and diagnostic support, rehabilitation support, eldercare), and industrial settings (e.g. automating warehouse workflows, automating production lines, supporting with safety and inspections). Other areas of interest are likely to include last-mile delivery, security, hospitality, construction, and agriculture.
Framework for AI Applications
As an early-stage investor, when we consider the most investable opportunities in the application layer of AI, we are guided by a few key beliefs and guiding principles, and we will walk through those and the framework that supports our investment decisions.
Domain specificity (vertical > horizontal)
Often, the starting point for defining preferences in the application space requires recognising the difference between vertical and horizontal applications. Vertical applications are specialised software solutions that are tailored to a specific industry, whereas horizontal applications are more generalisable and applicable across various industries. However, the lines can at times blur, and such categorisations typically exist to varying degrees and across a spectrum. Discussing companies in terms of being “more vertical” or “less horizontal” is though rather unnatural, and thus we prefer to discuss companies in terms of “domain specificity” and consider the following matrix.
The more an application is positioned towards the top right, the more domain specificity it has, and thus the more inherent the potential advantages for building a high quality product and scaling it with a repeatable approach to commercialisation. Whereas the more generalised an application is, the more likely it is to (i) compete with some of the largest incumbent tech platforms, and (ii) be at risk of being competed away by a general intelligence and advancements driven by foundation models.
Of course, the more domain specificity an application has, the more limited its total addressable market will be, so the sweetspot has to lie with those applications that combine specificity with large market opportunities.
Identifying domains ripe for AI transformation
Given a preference for applications that trend towards domain specificity, it is subsequently key to consider what characterises the domains in which we see (i) the most potential for AI transformation, and (ii) the greatest likelihood that that transformation can be delivered by a new startup. This leads us to guiding beliefs related to:
Value creation
Data
Incumbents
To elaborate, we seek opportunities that lie within the domains where we see:
(i) The highest potential for value creation in mission critical workflows
Improving the effectiveness and quality of output
Increasing efficiency and lowering cost
Or otherwise creating entirely new value (new offerings, revenue streams or business models)
Exactly how value creation is measured will vary depending on the application and use case, and may be more or less clearly tangible vs visionary. Given the challenges of standing out in enterprise sales, it’s essential (especially at this point in the overall adoption curve) that new applications can provide significant and tangible value to an organisation’s most mission critical workflows, measurable on the top/bottom line.
(ii) Data advantages
Domain-specific data that requires expertise to both access and work with
Difficult-to-access data
Perhaps due to closed systems, data sensitivity, data existing outside of the public realm
Advanced data-handling and infrastructure
Requiring working with various data sources and data types
Potential for a proprietary data flywheel within the product and its usage
Given a domain exhibits these kinds of characteristics, application builders have greater potential to build data moats and defensibility, while such a domain would also be harder to address for the general intelligence of foundation models.
(iii) A lack of technically capable incumbents
Domains still dominated by legacy players with low tech capability and low agility are much preferred to domains in which there is the strong presence of big tech platforms or several VC backed winners from the past 10 years
Technically savvy incumbents, with accumulated data, with customers and distribution, will often have the potential to integrate AI functionality into their offerings → the shift is not so cumbersome and the existing data and customers provide advantages for building better products. See for example the AI agents being introduced by large horizontal platforms such as Salesforce, Workday, and ServiceNow.
Overall, the basic game that is being played is that the innovation potential of a startup must be able to overcome the distribution power of an existing player.
As a note, we think this dynamic is evolving. Right now we are early in the AI platform shift and it’s particularly expensive for new startups to sell an emerging technology to immature buyers, bearing the cost of figuring out how best to package applications and build AI-native interaction design. When some of these elements have gained maturity, there may be an easier path for new startups to rapidly scale their applications and compete even against more technical incumbents — as we have seen with e.g. the shift to mobile, it often takes some years before the biggest winners emerge.
The “old” SaaS moats
For all the talk around AI applications regarding competitive advantages and defensibility related to models and data, it is important to acknowledge that the “old” SaaS moats still hold high relevance and remain amongst the key drivers of success.
(i) Product plays a huge role
Depth of understanding of enterprise processes, user behaviours, and workflows → in AI this also means finding the “right” positioning between human-in-the-loop and human-out-of-the-loop
Quality of UX/UI to provide a tailored, intuitive, streamlined experience
Depth of integrations with other apps and data
(ii) Frequency of interaction
Driving stickiness and retention
Generating more data to feed the flywheel
Providing input to inform product development and improvements → daily usage feeds product iterations far faster than weekly
(iii) Sales and distribution effectiveness → enabling repeatability
Clear ICP, buyer and user personas
Clear sales motion
Direct sales
Indirect sales via ecosystem of partners, integrators, resellers
Competitive business model and effective pricing
Great products alone do not win markets, and usually the brilliantly executed go-to-market strategy of an inferior product will overcome the mediocre commercial efforts of a better one. So the greater the clarity and understanding of all things that enable repeatable sales, the better.
The AI applications cheat sheet
Bringing this all together, we can summarise in one page a “cheat sheet” for guiding our view of AI applications. While there are no absolute or ultimate truths, each consideration guides us in further understanding the risks and opportunities related to a potential early-stage investment in the application layer.
Applications: where we’ve been investing
We invest actively in AI applications across a range of industries, and thus are agnostic in that sense. However, the preferences outlined in our framework have led us to spend some additional time and effort in a few spaces, including AI in industry, in healthcare, and in professional services. These domains are characterised by their specialised data and processes, an array of use cases lacking technically strong incumbents, and the range of mission critical workflows that can be greatly benefited by the application of AI.
Below, you see a mapping of the workflows and processes we believe AI has the potential to transform and provide great value towards, and where we believe that that value can be delivered by new entrants to the market. You will also see a number of our recent additions to the portfolio that relate to these domains.
Industrial and healthcare applications are also being greatly boosted by a number of the defining themes highlighted earlier — with cheaper hardware, edge AI, and multimodal capabilities enabling the usage of sensors, cameras, and other devices for things such as remote monitoring (of machine processes or patients), diagnostics (of production issues or illnesses), and various robotics applications supporting previously manual processes.
Industry
IPercept — redefining efficiency for industrial machines
Buddywise — real-time risk detection enabling industrial companies to build safer workplaces
Healthcare
MVision — precision radiotherapy treatment planning
Rely — internal developer portal providing visibility across the entire software delivery lifecycle.
Professional services
Ayora — AI for legal P&L management
Counsel — making legal simple.
Jimini — assisting in researching, analysing and drafting legal documents with unparalleled efficiency.
Retail
Dema — AI decision platform for e-commerce.
6. The Context
In order to comprehensively observe what is happening in data and AI, and foresee what is next, we have to consider the context around the technology, and the impact of key factors such as regulation, national strategies, the availability of talent, and access to capital. It is also important to acknowledge the impact that increased AI activity has on the world around us, and how our understanding of those effects should shape the way we build and invest in the technology going forward — here we look at the climate impact of AI as it is already somewhat measurable; in future analyses we’ll also aim to dive deeper into understanding further aspects such as the emerging social impact of AI, effects on the labour market, unequal access to technology, and potential for overall increased inequality.
During this section, we will take a closer look at these areas in order to understand how they are contributing to shape, inhibit, or enable developments. Given that the subject scope has the potential to be so broad, we at times shine a light on the global context, and at other times provide a more focused view on the European context.
Regulation
Around the world, national governments and international organisations are accelerating their efforts to create AI regulation. Some policymakers see it that they are fighting to stay ahead of the pace of technological development. And others, that they are rushing to keep up with it.
In theory, AI regulation aims to safeguard against potential risks and misuse, enable an ecosystem of rapid development and progress, and ensure that the overall impact on society is well-managed and net-positive. It is a difficult task to strike the balance of these aims, with restrictive policies finding their fit between being responsible and necessary versus constraining and anti-innovation, and enabling policies finding their fit between being progressive and supportive versus irresponsible and dangerous. All stances and opinions across these spectra can be heard loudly and passionately.
One of the biggest issues has been to establish who should shoulder the burden of responsibility, and thus where regulations should be targeted. In other words, should regulation be directed towards the builders of powerful AI models, or towards those that develop the applications to use those models in different settings? Leading AI researcher Andrew Ng and Yann LeCunn (Chief AI Scientist at Meta) have articulated this point well. Essentially, we are distinguishing between regulating a technology (e.g. powerful foundation models) and regulating applications (e.g. a recruiting automation tool connected to an LLM, or a personalised healthcare solution connected to an LLM). At its worst, regulating a technology would mean regulating research efforts, and creating obstacles for fast and open AI research. While a technology can be applied in many different ways to solve various problems, an application is a specific implementation of that technology designed to meet particular needs. Andrew Ng’s analogy is a perfectly good and clear one, so we will repeat it here:
“For example, an electric motor is a technology. When we put it in a blender, an electric vehicle, dialysis machine, or guided bomb, it becomes an application. Imagine if we passed laws saying, if anyone uses a motor in a harmful way, the motor manufacturer is liable. Motor makers would either shut down or make motors so tiny as to be useless for most applications. If we pass such a law, sure, we might stop people from building guided bombs, but we’d also lose blenders, electric vehicles, and dialysis machines. In contrast, if we look at specific applications, like blenders, we can more rationally assess risks and figure out how to make sure they’re safe, and even ban classes of applications, like certain types of munitions.”
Thus, safety is a property of applications, more than it is a property of technologies (or models). There are, of course, AI safety questions that need to be addressed at the model level, and the builders of advanced models must take responsibility with regards to topics related to training data, inherent biases, levels of transparency, and efforts towards alignment. But much of the safety depends on the context and way in which an AI model is applied. Thus, it is in recognising this distinction between technology and applications, and directing appropriate policy towards each layer of the AI stack, that AI regulation can be most clear, fair, and effective.
The last couple of years have really seen AI policy and regulation take centre-stage as a topic of heated discussion, and a prioritised agenda item for lawmakers. If 2023 was the year of creating plans, roadmaps, and aligning on vision, then 2024 can be seen as the starting point of action and implementation.
EU AI Act
In the same way that the EU was a first-mover in introducing data protection regulation in the form of GDPR, becoming the de facto global standard subsequently mimicked by regions all over the world from India to California, the EU has acted quickly to introduce its EU AI Act in the hope that it can again go some way towards shaping the way the world does business and develops technology.
In March, the European Parliament voted to pass the AI Act, with a final green light coming in May, before it came into force on 1st August. Regulators are getting themselves set up in order to enforce the law (a new AI Office has been established), while companies will have up to three years to comply with it.
The AI Act applies broadly to “AI systems” - covering traditional machine learning as well as generative AI - and will affect all firms seeking to do business within the EU, regardless of where they are based. At its core, the regulation defines a three-tiered risk classification of AI systems, as either unacceptable risk, high risk, or minimal risk, based on their intended use and the potential impact on individuals and society.
Unacceptable risk refers to AI systems that pose a significant threat to people’s safety, rights, or fundamental freedoms, and are therefore banned. They include systems that:
Use real-time facial recognition in public places
(The police would need to get court approval for specific purposes such as anti-terrorism, finding a missing person, or preventing human trafficking)
Use emotion-recognition technology at work or in schools
Create facial recognition databases by scraping the internet
Infer sensitive characteristics such as a person’s sexual orientation or political opinions
Deploy “social scoring” and classify people based on behaviour, socio-economic status or personal characteristics
Exploit vulnerable people or utilise cognitive behavioural manipulation to distort behaviour and impair informed decision-making
High risk refers to AI systems that pose a significant risk to people’s health, safety, or fundamental rights. To ensure their safe and ethical deployment, these systems are subject to stringent regulatory requirements. They include systems that relate to:
Healthcare
Education
Law enforcement
Human resources and recruitment
Public services
Administration of justice and democratic processes
Transport systems
Some of the obligations that providers and deployers of “high risk” systems must adhere to are:
Risk management across the whole AI lifecycle
Data governance and ensuring high-quality datasets for training, validation, and testing
Human oversight to minimise risks and ensure and ensure accountability
Maintaining detailed technical documentation
Ensuring that high standards are met for accuracy, robustness, and cybersecurity
Reporting energy consumption, resource use, and other impacts throughout the systems’ lifecycle
Minimal risk refers to AI systems that pose little to no risk to people’s rights and safety. As a result, these systems are subject to the least regulatory scrutiny and can be freely used, though firms are encouraged to follow general principles related to fairness, non-discrimination, and the use of human oversight. Examples of minimal risk systems may include:
Inventory management systems
Predictive maintenance
Personalised e-commerce recommendations
AI-enabled video gaming
Customer service chatbots
Regulating models
While the tiered risk classification addresses the application of technology towards different use cases, the AI Act also aims to regulate those firms developing the “general purpose AI models”, with different obligations depending on how powerful the model is considered to be.
All companies developing “conventional general purpose AI models” will need to maintain technical documentation showing how the model was built, publish a summary of the underlying training data, and prove that they respect copyright law.
Companies developing more powerful models, so-called “systemic risk general purpose AI models”, are subject to more stringent obligations, including needing to perform risk assessments and model evaluations, ensure high degrees of cybersecurity protection, undergo enhanced testing, and comply with reporting requirements such as any incidents of system failure.
The distinction between those models classified as “conventional” and those classified as “systemic risk” is made based on FLOPS - the “floating-point operations per second” measure of computational power that a model is capable of. The AI Act defines “systemic risk general purpose AI” models as those with more than 10^25 FLOPS, which includes models such as GPT 4, Gemini Ultra, and Mistral Large.
While there are currently not too many models meeting this classification (as of April earlier this year, Epoch AI estimated less than a handful), given the rapid pace of AI development and model scaling, it is likely that many more will fall under the regulation going forward. As a result, the AI Act retains the flexibility to adjust the threshold if the landscape sufficiently changes. Notably, in the US, the Biden Executive Order on AI sets the threshold at 10^26 FLOPs, which is a substantial increase in computational power, and likely equivalent to a 10x higher cost of model training.
So what a FLOPS-based threshold should be is clearly up for some debate. At the same time, whether such a measure makes sense or not is also a point of discussion, given that the quality of models that can be trained within this threshold is rapidly improving (e.g. with highly advanced smaller models), meaning that FLOPS may not be representative enough of model capabilities.
Big tech’s concerns and Europe losing out
In September, Mark Zuckerberg, Daniel Ek, Yann LeCun, and a number of technology leaders and researchers signed an open letter to the EU, asking for regulatory certainty in order for the region to not fall behind.
In accordance with claims that have long been raised, the signatories state that Europe has become less competitive and innovative, and risks falling further behind on AI, due to inconsistent regulatory decision-making - with, for example, interventions by the European Data Protection Authorities creating huge uncertainty around what kinds of data can be used to train models.
As a result of this inconsistency and uncertainty, the signatories claim that the EU is set to miss out on two cornerstones of AI innovation - namely developments in open models, and developments in multimodal models. This has recently been seen to come to reality, with Meta not releasing its latest Llama 3.2 multimodal models in the EU, and OpenAI not making its Advanced Voice Mode available in Europe. If European builders lose out on access to the latest open models, then they can only fall behind others based elsewhere who are not prohibited and can continue to develop faster.
Looking forward
Now that the basis of regulation is set, how, and how quickly, the EU continues to refine and shape it will be key to enabling European tech companies to thrive and compete as AI winners are built. It’s worth noting that the five-year term of the EU Commission which has led the development of the EU AI Act is now coming to an end, meaning that a new college of commissioners will be formed and tasked with the implementation and refinement of the regulation. It remains to be seen if this will represent an opportunity for fresh perspectives to continue to evolve the regulation, or if it will contribute to a bumpy ride in its enforcement.
National Strategy and Regional Policy
The policies of nation states will clearly impact the overall speed of technological development, where it takes place, and what kind of impact it will have. As we continue to proceed into this era of AI, governments around the world are tasked with setting out their strategies for seizing the opportunity, remaining internationally competitive, and ensuring that their societies benefit from new technology in both the near and longer term.
On a European level
The September publishing of Mario Draghi’s report into “The future of European competitiveness”, re-initiated calls for policymakers to ensure that the continent does not miss out on this AI platform shift. The report highlights the innovation gap between Europe and the US & China, and calls for
Annual funding of €750-800bn for EU projects
Strategic focus on innovation, and enabling European companies to become €100bn winners that remain European
Improved EU coordination, addressing policy and regulatory fragmentation
Seizing decarbonisation and the energy transition as an opportunity
Alongside the recent discontent from tech leaders regarding Europe’s approach to regulation, there is a growing feeling from some early-stage builders that they are much better off basing themselves in the US - given frontier innovation, access to more capital, more favourable regulation, better access to the latest AI models, and of course access to a large single market.
Evidently, there is work to be done to create the conditions that will enable Europe to thrive and be a relevant technological force in the coming years.
Comparing countries
Recently, a number of rankings have emerged, trying to compare countries in their approaches to AI and/or their potential to embrace it. Such rankings are of course highly complex and rather subjective, and thus very limited conclusions can be drawn from them, but they do make for an interesting point of departure in discussions around what can or needs to be done.
The Global AI Index looks at 83 countries and ranks them based on AI implementation (talent, infrastructure, operating environment), innovation (research, development) and investment (government strategy, commercial ecosystem). The 2024 ranking can be seen below.
While it’s unsurprising that the US and China sit at the top of the rankings, what is more interesting, and alarming for other nations and Europe as a bloc, is the size of the gap between those two at the top and the rest.
During the summer of 2024, the IMF published its AI Preparedness Index assessing the level of AI readiness across 174 countries, and considering factors including digital infrastructure, human capital and labour market policies, innovation and economic integration, and regulation and ethics.
This ranking does not paint the same extreme picture with regards to the frontrunners, likely because it focuses on ranking the ability to embrace and integrate the technology, rather than the ability to develop it, drive the innovation, and essentially own the value chain, as the Global AI Index addresses. According to the IMF ranking, much of western Europe is well-placed to embrace AI (Denmark scores 0.78, UK scores 0.77), alongside North America (US scores 0.77). While China lies a little behind with a score of 0.64. The big warning though is how AI could widen existing inequalities between wealthy and developing countries, and the IMF encourages efforts to open up access to state-of-the-art technology.
Recent national policies and actions
Countries all over the world are pouring billions of dollars into new domestic computing facilities for AI
Countries and regions setting regulation and large investment commitments to boost their computer chip competitiveness
The CHIPS Act in the US aiming to boost the US semiconductor industry, as well as Europe’s own EU Chips Act
South Korea unveiling a $19bn package of incentives to boost its chip sector
China’s $47bn semiconductor fund to ensure chip sovereignty
Governments seeking to attract big tech firms such as Microsoft, Google, and Amazon, to make multi-billion dollar investments in cloud and AI infrastructure in their countries, as seen e.g. in France and Sweden
Saudi Arabia allocating $100bn to invest in technology, including a massive $40bn fund to invest in AI, as it aims to become a global AI hub
With recent elections in France and the UK, and an upcoming election in the US, major economies are providing mandates to newly established leadership. The policies and actions of those governments will go some way to shaping who stands to win or lose the most in the years ahead.
10 national strategies to thrive in the AI era
In order to thrive in this era of AI - to enable technological development, economic growth, and widespread societal benefit amidst significant transformation - governments and regional blocs need to take assertive action rather than be asleep at the wheel. Here, we highlight a number of initiatives that we believe governments need to be acting upon in order to build positive momentum in this era of technological change and opportunity. The list is non-exhaustive, and some of these actions are more urgent than others, but to dive deeply into these topics is an exercise for another occasion.
Secure sovereign access to computing resources
Governments need to ensure that their countries are not left vulnerable by a lack of access, independence, or control when it comes to computing infrastructure. They need to work together with the private sector in order to ensure security across the entire value chain - from research and development, hardware production and supply, to data centres, AI models, and core tooling.
While not all of these components need to be addressed and secured on the national level - some may be better tackled across regions, trade blocs, or bilateral agreements - governments must have clear strategies for the independent initiatives and cross-border collaborations that can secure the necessary compute resources and capabilities across the AI stack.
This will enable a greater degree of influence and control towards national security (against foreign interference and cyberattacks), data sovereignty (better ensuring that data laws and regulations are adhered to), economic independence (not overlying relying on foreign technology providers), and resilience (to recover core infrastructure in the event of a crisis or disaster).
Enable the energy transition
As we have seen throughout this report, there is extreme demand for increased compute resources in order to power AI development and applications, and this compute consumes huge amounts of energy resources (see more in the climate impact section). Significant investment is required to prepare the energy grid for AI power demand, and, as AI leadership may be strongly correlated with energy prices, it is essential that governments deliver on strategies to enable low cost, stable, and renewable power.At the same time, we have seen that computational efficiency can be improved and energy consumption reduced by developments in data centre infrastructure, chip architecture, models, and software tooling. Governments should be intentional in funding research efforts, and incentivising private sector actors, that have the potential to improve energy efficiency in relation to AI and compute.
Beyond the specific challenges of AI power demand, we recognise that much of the broader energy transition is a financing problem more than it is a technology problem. Solutions such as batteries, solar, and heat pumps exist but often with high upfront costs, even if their lifetime costs are lower compared to fossil alternatives. Governments need to be consistent in their policy and long-term in their thinking, so as to best encourage companies and households to also think long-term and make sensible energy choices. At the same time, governments should encourage the private sector to develop and provide financing solutions that enable businesses and households to transition to renewables, so that upfront cost does not get in the way of delayed benefit.
Incentivise investment
In order to fuel technological innovation, long-term economic competitiveness, and ensure homegrown category-leading AI companies, governments must ensure that their policies incentivise increasing amounts of investment in the necessary high-risk high-innovation technologies and companies.
As we cover in the “Access to capital” part of this report, European asset managers still allocate only half as much towards venture capital as their US peers, while pension funds in particular are allocating very little to the asset class. This indicates the potential in Europe to unlock more capital that can fuel this wave of AI innovation. And given the scale of AUM, even miniscule percentage increases in amounts allocated towards venture capital have the potential to drive significant investment and innovation. Thus, government policies that aim to mobilise institutional capital can have an outsized impact. Examples of policies may be public-private partnerships (such as the Tibi Initiative in France), tax incentives, regulatory reforms, or government support for fund-of-funds structures.
Attain and retain talent
It’s remarkable people that force breakthroughs at the forefront of research and technology, and that start and build category-leading tech companies. The best and most ambitious talent will go where they are empowered with the best chance of succeeding and having the large-scale impact they believe they can achieve. Thus, competitiveness starts from creating the conditions that best attract and retain world-class talent. These efforts must focus both on research and academia (increasing funding to universities and research organisations), as well as the private sector (increasing investment, incentivising entrepreneurship, creating the conditions for companies to compete).
Regulate with clarity and agility
We cover the current state of regulation in the EU elsewhere in this report, where we also acknowledge the difficult balancing act that policymakers have in setting regulation that both safeguards against legitimate risks and enables a fast pace of innovation. The speed of technological development - with improving model capabilities and new applications - means that the goalposts are continuously moving, but the picture is also becoming increasingly clear. As a result, regulators need to be open to reassessing their frameworks - such as where to set FLOPS-based thresholds, how to distinguish between different risk levels, how data should be handled, or what mitigating measures are in fact reasonable and effective. This openness to refining regulation of course needs to be managed against the need to provide clarity and consistency so that companies and individuals can most easily plan and navigate. Again, another part of the difficult balancing act, but another key factor in laying the overall foundation for economies to thrive and seize the AI opportunity.
Prepare people, broadly educate, and facilitate dialogue
Technological change can be daunting at the best of times, as uncertainty breeds concern. In the context of AI, this has been exacerbated by media scaremongering and discussions about risks that are at times perhaps extreme and distracting. This risks creating an environment of fear and ignorance, and leading individuals to widely reject new technology rather than interact with and embrace it → resulting in many being left unprepared when its implementation and impact starts to be more widely felt.
Governments should work to build a positive (while of course objective) narrative and educate individuals regarding the potential of AI to improve people’s economic and life outcomes through increased earnings, and improved healthcare, education, and critical infrastructure. They should engage and consult citizens’ assemblies to identify fears and priorities, in order to build public trust and inform decision-making, and should encourage employers and employees to discuss the potential impact of AI in the workplace, in order to address fears related to job safety.
Integrate AI within government
Governments should lead by example and integrate AI across government - working to successfully implement the technology, as well as to build a culture of progressive thinking towards it, such that government operations can increasingly become technology-first, and AI-enabled. Not only will this serve to improve the efficiency and effectiveness of public services and administration, but it will also provide greater insight and experience for those politicians and civil servants tasked with steering AI policy across the private sector and society.
Update the school curriculum and integrate AI within schools
The school curriculum should be updated to emphasise, test, and evaluate the core skills that are required to make the most of AI tools and prepare for an AI-native job market. This includes focusing on
(i) problem solving related to analysis and logical thinking, creative thinking, and quantitative and statistical skills.
(ii) collaborating and judgement in the form of task delegation, machine understanding, and evaluation.
AI should also be seen as a great opportunity to accelerate every child’s learning, as we move from a one-size-fits-all approach to teaching towards fully personalised and highly interactive education. This opportunity should be embraced, and programs should be established that guide and incentivise schools towards engaging with new tools, and support them in their safe implementation.
The countries that more quickly and more deeply integrate AI into the delivery of education will see significant compounded effects in the knowledge and skills of their young people and labour market inflow.
Be predictive and proactive in forecasting job market trends
Fund collaborative efforts across academia and industry that seek to understand (i) the unique characteristics, strengths and weaknesses of the economy, (ii) the changing nature of different industries, and (iii) the forefront of AI technology and its application. By putting significant resources towards this, governments can better predict how and where AI may disrupt the workforce, as well as where opportunities for job creation may emerge, so that actions taken can be proactive more than reactive, and lead to a net-positive effect on the labour market.
Become agile and generous in training and retraining
To the extent that governments can be somewhat successful in point (9) above and identify changes within the labour market, as well as inherent strengths that the economy may have to further capitalise on, the next step is to take quick and meaningful action. The faster that training programs can be implemented to support workers in upskilling and seizing productivity gains of AI, the more advantageous to national competitiveness and economic growth. While the faster that retraining programs can be implemented to support those who suffer job losses as a result of technological change, the softer the landing for those individuals, and the cheaper it will be in the long run as those workers more quickly re-enter the workforce.
These efforts will require various forms of public sector support as well as public and private sector collaboration, as governments enable and incentivise companies to invest in upskilling and retraining.
[Bonus] Find the “Home PC” reform of AI
Over the past couple of decades, Sweden has established itself as a strong entrepreneurial tech hub, home to global category leaders such as Spotify, Klarna, King, Truecaller, and Kry. There are many reasons and contributing factors for why this small Nordic nation has been able to achieve such repeatable success, but one factor often referred to is the “Home PC Reform” policy of the late 1990s. This policy allowed employees to acquire a computer at less than half the retail price, through a tax-exempt arrangement provided by their employers. The reform had a significant impact on computer adoption in Sweden, as the number of households with at least one computer doubled in the first four years of the subsidies. The policy emphasised access to computers rather than just knowledge about them, and has been credited with contributing to Sweden becoming a leading nation in terms of IT literacy, and enabling a digital-native generation. This in turn helped to lay the foundation for the tech talent and founding of some of the aforementioned unicorn successes.
The “Home PC” reform was introduced at a time when the internet and computers were quickly changing the nature of work and life at home. Now, as AI is set to do the same, we ask “what is the Home PC reform of AI?” Can similar public-private schemes subsidise and provide households with greater access to AI tools? Or, more broadly, what policies can contribute to increased AI access and literacy such as to enable a generation of technically capable workers, innovators, and founders of AI category leaders.
Access to talent
It is clear that the US remains vastly superior to Europe and the rest of the world when it comes to the quantity of top AI talent. This is evidenced by the dominance of US universities at the forefront of AI research, and the dominance of US Big Tech firms in hiring engineers.
However, Europe’s AI talent foundation is strong, with positive momentum that will serve the ecosystem well going forward. We’ll explore each of these points in a little more detail below, but in short, with strong AI research institutions, a growing presence of leading tech and specifically AI firms, and an ability to retain much of the best AI talent and be a net importer of tech personnel, Europe has an increasingly strong flywheel for creating category-leading companies across the data and AI stack.
Europe’s AI talent flywheel is just starting to turn
European tech has been growing from strength to strength over the past couple of decades, and it is well established that each unicorn success contributes to the next wave of founders creating new companies. Founder mafias have emerged from companies such as Spotify, Revolut, Skype, Wise, and Klarna and given birth to dozens of new startups. As Atomico highlighted in their 2023 State of European Tech Report, this talent flywheel of unicorn alumni is accelerating, with nearly 9,000 companies having been created by alumni of European exited unicorns that were founded during the 2000s, almost 50% more than the number created by alumni of unicorns founded in the 1990s. Looking at the trend of the most recent cohort of unicorns founded in the 2010s, the pace only appears to be picking up.
When it comes to the specifically AI talent flywheel, we’re at an earlier stage but the momentum is certainly picking up. Large US tech firms such as Google and Meta have had established AI research presence in Europe for some time, while DeepMind, having been founded in London in 2010 has long been the jewel in the crown of the European AI ecosystem. It’s clear that these firms have served to provide the foundation for talent fueling the current wave of AI startup activity. A recent report by Accel and Dealroom, analysing 221 GenAI startups from across Europe and Israel, found that 25% of those companies have one or more founders who have worked at Amazon, Apple, DeepMind, Facebook, Google or Microsoft, and this increases to 38% when looking at the top 40 companies in terms of funding, and even further to 60% when looking at the top 10.
We expect that this flywheel is only just getting started as:
US presence continues to grow with leading AI players such as Open AI and Anthropic (building models), and Scale AI and CoreWeave (providing enabling tooling and infrastructure), only recently establishing significant European offices
Europe continues to establish its own cluster of companies operating at the frontier of developing AI models or serving AI to enterprise, with the likes of Mistral, Aleph Alpha, and H
European leaders continue to emerge across the application and enabling layers of the stack - companies such as ElevenLabs, Synthesia, Wayve, Hugging Face, Poolside, and Parloa
These positive trends are creating the presence of more AI talent factories, and more factories will in turn lead to a higher volume of increasingly capable AI founders.
Disclaimer: While we fully believe in these positive dynamics over time, in the short-term the presence of relatively few, extremely well-funded companies is likely to pose a significant challenge to the rest of the startup landscape fighting for top AI talent. Anecdotally, this is already playing out in Paris, where the likes of Mistral, H, and Poolside represent formidable hiring competition for companies that have a fraction of their budgets.
The role of universities
When looking at which universities are contributing the most to AI research and the development of frontier models, the US clearly leads the way with Stanford, Carnegie Mellon, UC Berkeley, and MIT the biggest contributors. Europe does, though, have three universities out of the top 12 global contributors to notable AI models over the past 10 years, with University of Oxford, Technical University of Munich, and ETH Zurich each featuring.
It is also clear that these academic institutions are just as essential to providing the foundation for Europe’s AI talent flywheel. Accel and Dealroom’s report illustrates the highly educated and rather academic nature of GenAI founding teams, with 38% of the companies analysed having at least one founder who holds or has held a position at an academic institution. This increases to 55% when looking at the top 50 companies in terms of funding, and even further to 80% when looking at the top 10.
When it comes to where the founders of GenAI companies are educated, Cambridge University, École Polytechnique, Imperial College London, University College London, and Oxford University lead the way, together educating one-third of GenAI founders in Europe.
Europe is a net importer of tech talent and is continuing to retain more of its top AI talent
It is often proclaimed that Europe is suffering a brain drain of tech talent, as people flock to join US companies or build in the US instead. Certainly, this is a sentiment and concern that most within the European venture scene are familiar with, however, there are a few data points that can potentially add nuance to the discussion and provide some optimism.
Atomico’s 2023 State of European Tech report shows how Europe continues to be a net beneficiary of talent movement, with 10k+ net new people joining the European tech scene during 2023.
While this inflow supports the tech ecosystem as a whole, Europe’s ability to build category-leading AI companies relies on the rate at which it can attract and retain world class data scientists and AI engineers. Over the past ten years, the US has been dominant in attracting AI talent from the rest of the world - powered by the growth of the likes of Amazon, Apple, Google, Microsoft, Meta, and Nvidia, the US has attracted and retained twice as much top AI talent as it has supplied to the rest of the world. However, as highlighted by the latest State of AI Talent 2024 report by research company Zeki, this trend appears to be slowing.
This shift in momentum is being driven by a combination of:
More “national champions” in other countries managing to retain their talent
The attractiveness for AI talent to leave Big Tech - seeking the opportunity to build something new and disruptive, while being compensated by significant equity and potential upside in highly valued VC-backed AI startups
Factors related to politics and welfare steering some talent towards Europe over the US
Access to Capital
A vast and well-functioning market of early and growth-stage capital is essential for funding and fueling the frontier companies emerging across the different layers of the AI stack. Overall, the picture for Europe is positive.
Data from Pitchbook shows how VC and growth funding in Europe has been growing over the past decade, and although down compared to the highs of 2021 the trend remains encouraging, particularly in comparison to US funding volumes. VC and growth funding in Europe is now at ~40% of the US, compared to only 16% as recently as 2015.
When it comes to AI funding, though, the US remains way ahead, with the San Francisco Bay Area clearly the undisputed leader in AI tech and funding dollars, as shown by Crunchbase data. During 2023, more than 50% of all global venture funding for AI-related startups went to companies headquartered in the Bay Area. This figure is heavily influenced by mega-rounds in companies such as OpenAI, Inflection AI, and Anthropic, and the SF share falls to 17% when looking at the number of rounds rather than total value. Dealroom also shows that since 2022, the US has invested 3x more than Europe in AI as a whole, and 7x more in generative AI. Despite this, huge funding rounds this year in European companies such as Wayve (UK, $1.05bn), Mistral (France, $645m), H (France, $220m), and Aleph Alpha (Germany, $500m) suggest that there exists the willingness and ability to heavily back European AI firms too.
The foundation is well set in Europe for even higher levels of investment, Atomico’s State of European Tech 2023 highlights the level of dry powder (the amount of committed but unallocated capital that funds have at hand to deploy) in Europe as having risen to $58bn for VCs, and $108bn when also including growth funds. Back in 2013, this number was just $14bn for VCs, and $23bn when including growth funds. Thus, European venture funds currently sit on by far the largest pool of deployable capital that the continent has ever seen, which should be encouraging for both the founders of startups, as well as the earliest backers of them taking the initial investment risk.
Atomico's report suggests that there exist significant opportunities for policymakers to work with asset managers to unlock even more funding for European tech. The report finds that European asset managers allocate 8% of assets under management (AUM) to venture capital, compared to 16% allocated by US asset managers. While pension funds continue to allocate very little to the asset class - in 2022, just 0.01% of pension fund AUM (which stands at $7.8 trillion) was invested into European VCs. With these numbers suggesting that there exists potential to unlock more capital to support technological innovation, a number of policies are aiming to facilitate and incentivise greater institutional investment in VC. For example, the Tibi 2 initiative in France, building on the success of the previous Tibi initiative, and aiming to attract up to €7bn of institutional capital into early-stage and deep tech investments.
Climate Impact
The impact of AI on the climate started to gain more attention towards the end of last year, when estimates started to show that making an image with generative AI uses as much energy as charging your phone, a ChatGPT query needs nearly 10 times as much electricity to process as a Google search, and Google’s AI-powered search may be 30 times more energy intensive than a standard Google search. While there has since been development in models and computational efficiency, there is no doubt that the compute required to run AI is energy intensive. And, as we covered earlier in this report when looking at hardware, demand for AI computing power is skyrocketing, as firms train larger and larger models, and speed and volumes of inference also increase. In Google’s latest environmental report, the firm shared that its emissions had gone up 48% over the past five years, largely driven by increased efforts related to AI. While some claim that the data centre emissions from big tech firms may be up to 7.62 times higher than those firms officially report.
Data centre power demand is estimated to grow 160% by 2030, according to Goldman Sachs Research. This will see data centres worldwide go from consuming 1-2% of total power to 3-4% (in the US this has been estimated to be as high as 8%), with developed economies experiencing the kind of electricity growth that hasn’t been seen in a generation → and a doubling of data centre carbon dioxide emissions as a result.
Alongside the growing carbon footprint is the issue of AI’s enormous water footprint. In its 2022 environmental report, Microsoft disclosed that its water consumption increased by 34% YoY, to nearly 1.7 billion gallons (or more than 2,500 Olympic-sized swimming pools), largely driven by its AI research activities. When xAI’s “world’s largest supercomputer” gets up to full capacity, it is estimated it will need a million gallons of water per day. Researchers at UC Riverside forecast that in 2027, global AI demand may be accountable for 4.2-6.6 billion cubic metres of water withdrawal, which is equivalent to about half of the UK’s annual water withdrawal. This has the potential to pose very real and pressing challenges, as freshwater scarcity is already a growing issue as a result of depleting water resources and outdated water infrastructure.
Companies building solutions to AI’s climate impact
We expect to see a growing number of companies in Europe building solutions to address the different parts of the environmental impact of AI, in areas such as:
Sustainable data centres powered by renewable energy, such as NexGen Cloud (UK), and evroc (Sweden)
New innovative approaches to cooling and energy efficiency within data centres
New more efficient chips, using photonics or other technologies, such as Black Semiconductor (Germany), and Lumai (UK)
Chips optimised for inference and the running of AI models post-training, such as Fractile (UK)
Software that enables developers to design their AI systems to train models faster, and get GPUs running more efficiently, such as FlexAI (France)
Transforming energy infrastructure
However, increased power demand will require significant investment in energy infrastructure. Goldman Sachs estimates that, as a result of the expansion of data centres and an acceleration of electrification, Europe’s power demand could grow by 40-50% between 2023-2033. Data centre power demand will predominantly rise in two archetypes of European countries:
Those countries with cheap and abundant power from wind, hydro, solar, and nuclear, such as the Nordics, Spain, and France
Those countries with large financial services and tech firms, and where tax breaks and incentives will be offered in order to attract data centres, such as the UK, Ireland, and Germany
Europe, though, has the oldest power grid in the world - fifty years old on average, compared to forty years old in North America, and twenty years old in China. As a result, Europe faces additional challenges compared to other regions when it comes to preparing the energy system for AI, and providing the AI data centre industry with what it needs, which in short is:
Inexpensive electricity costs - given the immense amount of power to be consumed, particularly as inference needs compound over time
Stability and robustness in the energy supply chain - safeguarded against geopolitical and weather disturbances
Power generation with a low carbon intensity - providing huge amounts of renewable energy
Thus, it will require huge investment in order to keep new data centres electrified. Up to €850 billion may need to be invested in renewable energy such as solar and wind, as well as up to €800 billion in spending on energy transmission and distribution. As outlined by Sequoia, AI will catalyse an energy transformation, and, beyond the rise of renewables, a resurgence in nuclear energy will be one of the long-term effects of the AI wave. This can already be seen amidst recent activity from big US tech players, with Microsoft agreeing an $800m per year deal to reopen the Three Mile Island nuclear power plant, Oracle revealing a data centre design powered by three small nuclear reactors, and Amazon announcing efforts to recruit nuclear engineers.
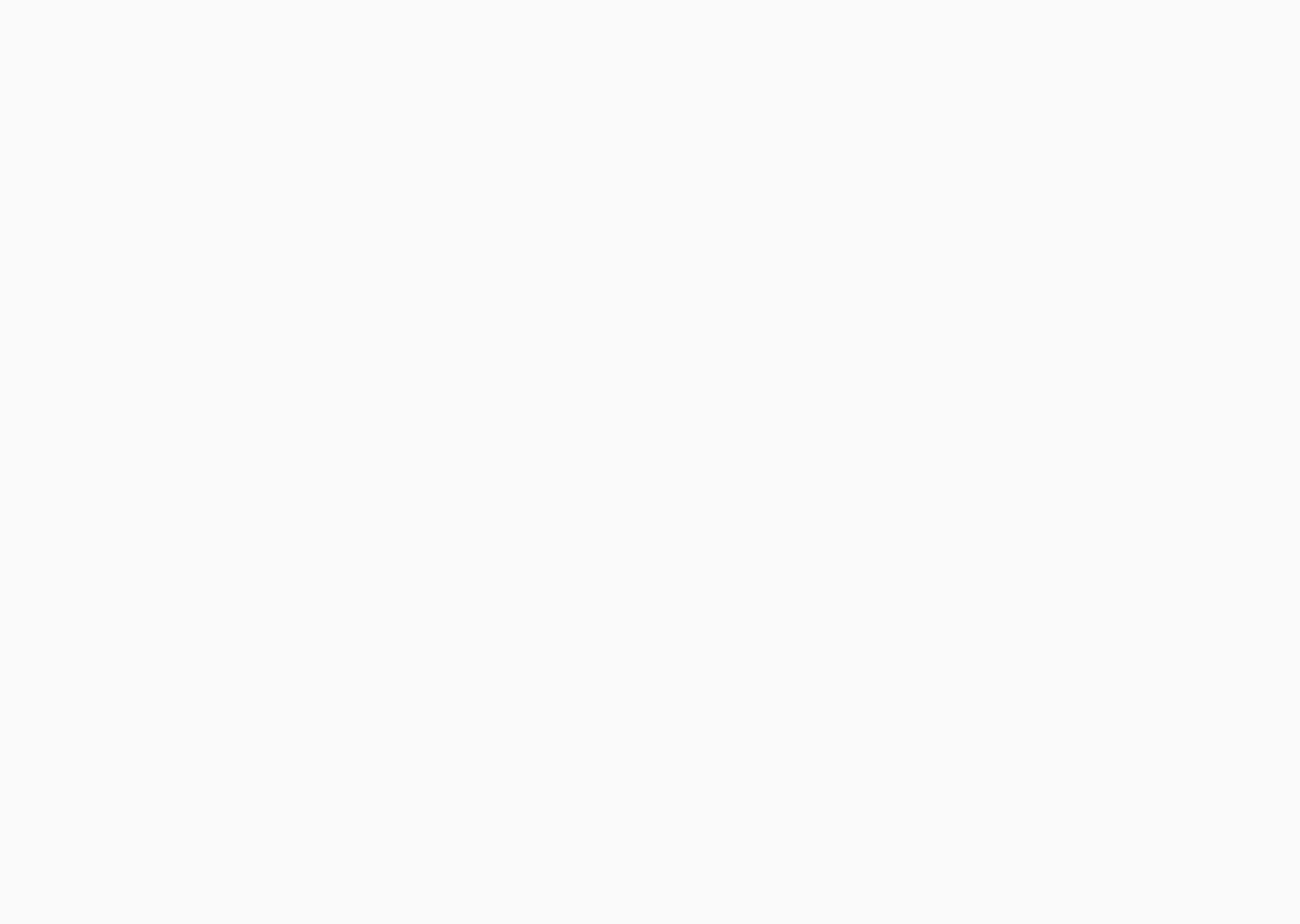
Contributors
The 2024 What’s Next in Data and AI report has been created in collaboration with BCG, with expert input from:
Andreas Lundmark — Managing Director and Partner, BCG X
Johan Öberg — Managing Director and Senior Partner
Christian Jacobsson — Principal, BCG
Daniel Sack — Managing Director & Partner, BCG X
Leonid Zhukov — VP Data Science BCG X, Director of BCG Global AI Institute
Tom Martin — Associate Director, BCG
Ronny Fehling— Partner and Director Generative AI, BCG X
Eugene Hayden — Tech Innovation, BCG
Kirsten Rulf — Partner and Associate Director, BCG
Jonathan Van Wyck - Managing Director and Partner, BCG
Niels Degrande — Principal AI Engineer at BCG X